Automated essay scoring (AES) uses AI and machine learning to evaluate essays quickly and consistently, making it ideal for large-scale assessments. It analyzes grammar, organization, and relevance using technologies like NLP and deep learning models. AES systems, such as IntelliMetric, handle high volumes efficiently, reducing grading time by up to 95%. While accuracy rates with human graders reach 85-90%, challenges remain in assessing creativity and nuanced arguments. Ethical concerns, like algorithmic bias, require ongoing attention. Combining human and machine scoring enhances fairness and reliability. Exploring further reveals how AES balances efficiency with the need for equitable, high-quality assessment.
Definition and Evolution of Automated Essay Scoring
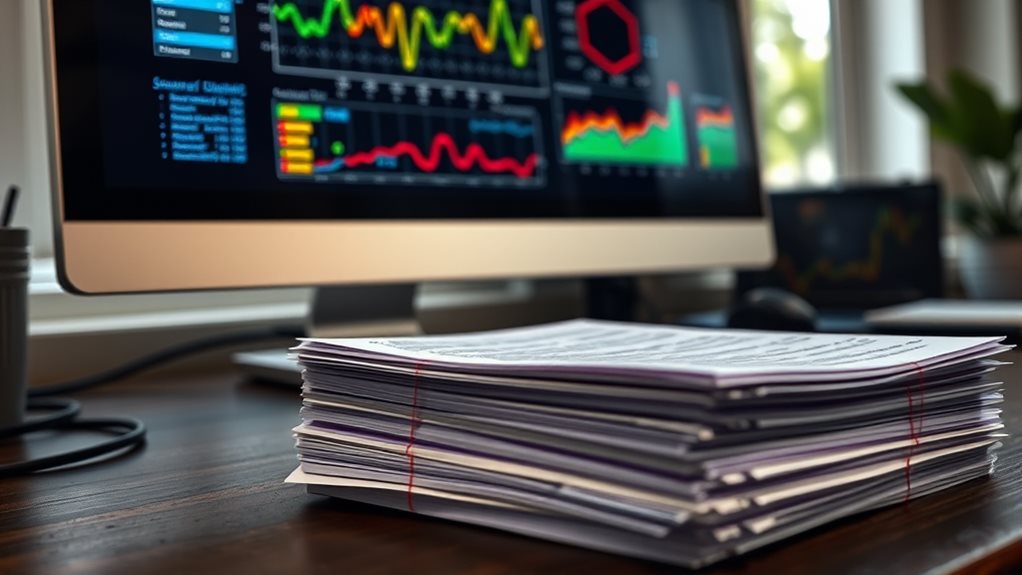
Automated essay scoring (AES) is a game-changer in the world of education, and if you're not paying attention, you're missing out on one of the most transformative technologies in assessment.
At its core, AES uses advanced algorithms to evaluate essays, providing consistent, objective, and efficient grading.
But let's break it down further—because this isn't just about replacing human graders; it's about revolutionizing how we assess writing at scale.
The evolution of AES is a fascinating journey.
It all started back in 1966 with the Project Essay Grader (PEG), a pioneering system that relied on simple pattern matching to evaluate essays.
While PEG was groundbreaking for its time, it was just the beginning.
Fast forward to today, and AES systems like the Intelligent Essay Assessor (IEA) are leveraging deep learning models to analyze not just grammar and spelling, but also style, coherence, and even the depth of content.
These systems are trained on massive datasets, allowing them to identify patterns and nuances that even experienced human graders might miss.
Here's why this matters to you:
- Efficiency: AES can grade thousands of essays in minutes, freeing up educators to focus on teaching rather than grading.
- Consistency: Unlike human graders, who might be influenced by fatigue or bias, AES provides uniform scoring across all submissions.
- Scalability: Whether you're dealing with a classroom of 30 students or a national standardized test, AES can handle the volume without breaking a sweat.
But let's not sugarcoat it—AES isn't perfect.
Early systems struggled with understanding context and creativity, often penalizing unconventional but valid writing styles.
However, modern AES systems are addressing these limitations by incorporating more sophisticated natural language processing (NLP) techniques.
For example, they can now detect subtle differences in argument quality, identify logical fallacies, and even assess the emotional tone of an essay.
The driving force behind AES development? The need for fair, efficient, and scalable assessment solutions.
As educational institutions face increasing pressure to evaluate larger numbers of students while maintaining high standards, AES offers a practical solution.
And with ongoing research focused on improving accuracy and fairness, the future of AES looks brighter than ever.
Key Technologies Behind Automated Scoring Systems
Automated Essay Scoring (AES) systems rely on a suite of advanced technologies to evaluate essays with precision and efficiency. Let's break down the key technologies that power these systems and how they work together to deliver accurate results.
Latent Semantic Analysis (LSA)
LSA is a cornerstone of many AES systems. Unlike traditional keyword matching, LSA dives deeper into the meaning and context of words. It analyzes the relationships between terms and concepts within an essay, allowing the system to understand the semantic content rather than just surface-level word usage.
For example, if you write about "climate change" and mention "global warming," LSA recognizes these as related concepts, even if the exact phrases differ. This technology ensures that the system evaluates the essay's coherence and depth of understanding.
Artificial Intelligence and Natural Language Processing (NLP)
AI and NLP are the brains behind systems like Pearson's Intelligent Essay Assessor (IEA). These technologies enable the system to assess not just grammar and spelling but also the organization, clarity, and relevance of your content.
For instance, if your essay lacks a clear thesis statement or jumps between ideas without transitions, the system flags these issues. NLP also helps the system understand nuanced language, such as idiomatic expressions or complex sentence structures, ensuring a more human-like evaluation.
Machine Learning
Machine learning algorithms are trained on vast datasets of human-scored essays, allowing AES systems to improve over time. The more essays the system processes, the better it becomes at predicting scores that align with human graders.
For example, if you submit an essay with a strong argument but weak evidence, the system can identify this pattern based on its training data and provide feedback accordingly. This adaptability makes machine learning a critical component of modern AES systems.
Statistical Approaches
Early systems like Project Essay Grader (PEG) relied heavily on statistical methods to evaluate writing characteristics. These approaches analyze patterns in your writing, such as sentence length, word variety, and syntactic complexity.
While these metrics might seem basic, they provide valuable insights into the technical aspects of your writing. For example, a high word variety score might indicate a rich vocabulary, while consistent sentence length could suggest a well-structured argument.
Hybrid Scoring Models
Some AES systems, like e-rater, combine automated scoring with human evaluation through a "Continuous Flow" approach. This hybrid model ensures that the system benefits from the speed and consistency of automation while maintaining the nuanced judgment of human graders.
For instance, if the automated system detects an unusual writing style or a highly creative argument, it can flag the essay for human review to ensure fairness and accuracy.
- LSA: Analyzes meaning and context, not just keywords.
- AI and NLP: Evaluates grammar, organization, and content relevance.
- Machine Learning: Improves accuracy over time with training data.
- Statistical Methods: Assesses technical writing characteristics.
- Hybrid Models: Combines automation with human judgment for optimal results.
Benefits of Automated Essay Scoring in Education
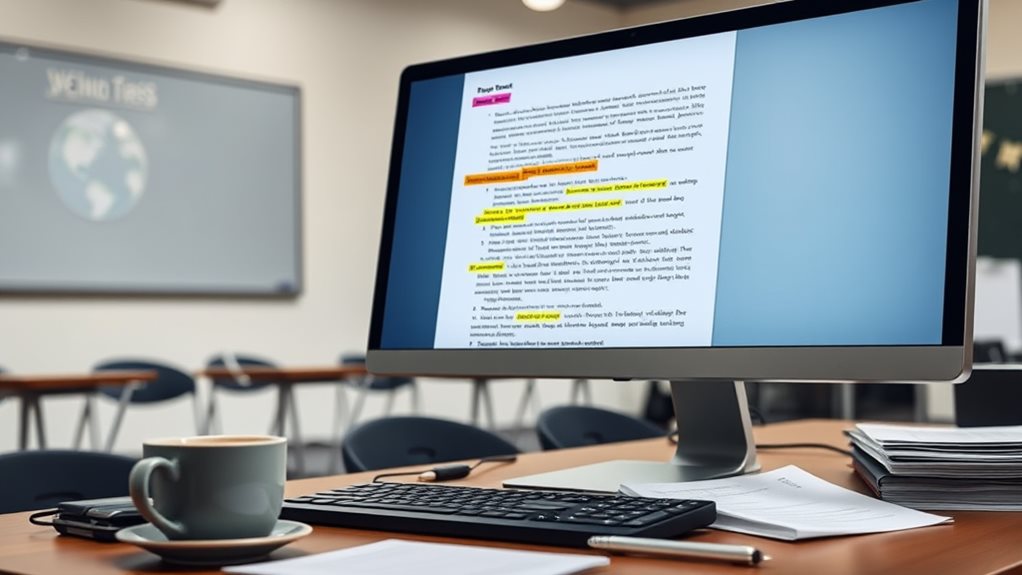
Automated Essay Scoring (AES) is revolutionizing education, and if you're not leveraging its benefits yet, you're missing out on a game-changing tool. Imagine cutting grading time from 10 minutes per essay to just 30 seconds. That's not a hypothetical—it's what systems like IntelliMetric deliver.
For educators and institutions, this efficiency isn't just a luxury; it's a necessity in today's fast-paced academic environment.
Here's why AES is a must-have in your educational toolkit:
– Efficiency: AES slashes grading time dramatically.
EssayGrader, for instance, claims a 95% reduction in grading time. That means you can assess hundreds of essays in the time it used to take to grade a handful.
- Cost-Effectiveness: Large organizations like the College Board and ACT have already adopted AES because it's a financially savvy solution. It reduces the need for human graders, saving both time and money.
- Consistency: Human graders bring bias, fatigue, and subjectivity to the table. AES eliminates these issues, ensuring every essay is scored against the same rigorous standards.
But the benefits don't stop there. AES also delivers timely feedback, which is critical for student growth.
Systems like EssayGrader provide immediate insights, allowing students to learn from their mistakes while the material is still fresh. This immediacy is something traditional grading methods simply can't match.
And let's talk about scalability. AES doesn't just grade essays—it evaluates multiple writing traits, from grammar and organization to development and coherence.
This comprehensive feedback gives students a clearer picture of their strengths and areas for improvement, something that's nearly impossible to achieve at scale with manual grading.
If you're still on the fence, consider this: AES isn't just a tool; it's a solution to some of the most pressing challenges in education today. It's time to embrace the future of assessment and unlock the full potential of your students—and your time.
Challenges and Criticisms of Automated Scoring
You need to understand that despite their growing popularity, Automated Essay Scoring (AES) systems face significant challenges and criticisms.
These systems often struggle to capture the nuanced aspects of writing, such as creativity, critical thinking, and argumentation.
Instead, they tend to focus heavily on surface-level features like grammar, syntax, and word count. This can lead to a mismatch between what the system evaluates and what truly matters in assessing writing quality.
When you consider high-stakes assessments, the fairness and validity of AES systems become critical concerns.
For example, think about diverse student populations or those with unique writing styles. Research shows that these systems may not handle variations in dialects, cultural expressions, or unconventional but effective writing techniques well.
This raises questions about whether AES can provide equitable evaluations for all students.
Studies comparing AES scores to human ratings have revealed inconsistencies.
For instance, a Pennsylvania study found that IntelliMetric sometimes showed higher agreement rates among its own scores than among human raters in certain dimensions. This discrepancy highlights the limitations of AES technology and its inability to replicate the nuanced judgment of experienced educators.
Here's what you should know about the key issues with AES systems:
- Overemphasis on surface features: Grammar and mechanics are prioritized over deeper meaning and overall essay quality.
- Limited construct representation: AES may not fully capture the writing construct, leading to incomplete evaluations.
- Potential bias: These systems may disadvantage students with unique writing styles or linguistic backgrounds.
- Inconsistent performance: Studies show AES can disagree with human raters, raising reliability concerns.
If you're relying on AES for assessments, you must weigh these challenges carefully. While the technology offers efficiency and scalability, it's not yet capable of replacing the depth and adaptability of human evaluation. For now, combining AES with human judgment may be the most effective approach to ensure fair and accurate writing assessments.
Accuracy and Reliability of Automated Systems
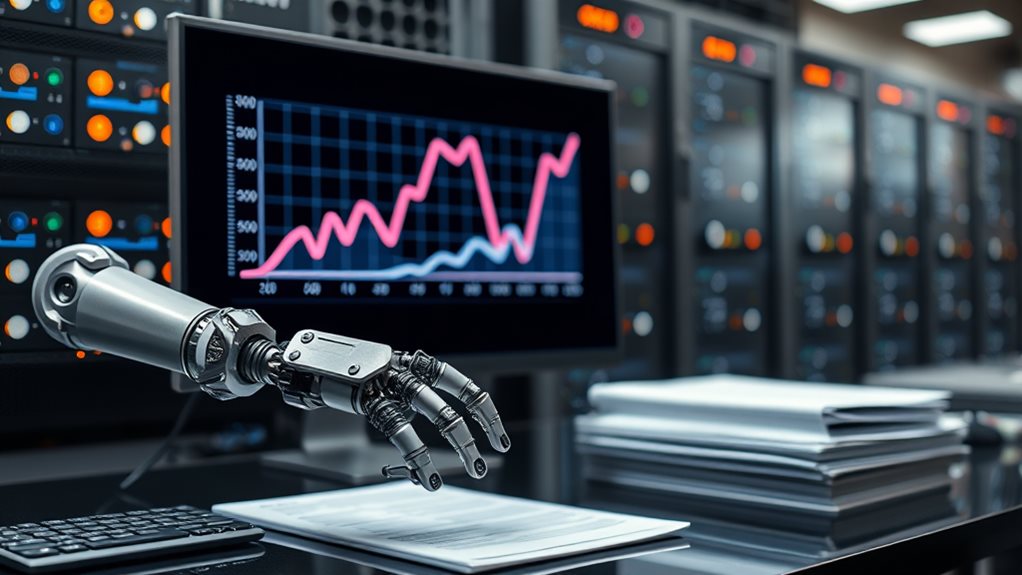
Automated essay scoring systems have come a long way, but their accuracy and reliability remain hot topics. If you're considering using these tools, you need to know exactly what they can—and can't—do. Let's break it down so you can make informed decisions.
How Accurate Are Automated Scoring Systems?
Automated systems are designed to mimic human grading, but they're not perfect. Studies show that these systems can achieve agreement rates with human graders of around 85-90%. That's impressive, but it also means there's still a 10-15% margin of error.
For example:
- Consistency: Automated systems excel at consistency. They don't get tired, bored, or biased, which means they can score thousands of essays with the same criteria every time.
- Complexity: Where they struggle is with nuanced writing. Subtle arguments, creative phrasing, or unconventional structures can trip them up.
If you're using these systems for high-stakes assessments, that 10-15% discrepancy could be a dealbreaker. But for formative assessments or practice essays, they're a game-changer.
Factors That Impact Reliability
Not all automated systems are created equal. Their reliability depends on several factors:
- Training Data: The quality and diversity of the essays used to train the system. A system trained on a narrow dataset will struggle with diverse writing styles.
- Scoring Rubrics: Clear, well-defined rubrics improve accuracy. Vague or overly complex criteria can lead to inconsistent scoring.
- Essay Length and Complexity: Shorter, simpler essays are easier to score accurately. Longer, more complex essays push the system's limits.
For instance, a system trained on high school essays might falter when grading graduate-level work. You need to match the system's capabilities to your specific use case.
How to Maximize Accuracy
If you're relying on automated scoring, there are steps you can take to improve results:
- Combine Human and Automated Scoring: Use automated systems for initial scoring, then have human graders review borderline cases or high-stakes essays.
- Calibrate the System: Regularly update the system with new training data to keep it aligned with your grading standards.
- Set Clear Expectations: Ensure students understand the scoring criteria so their writing aligns with what the system can evaluate effectively.
For example, if you're using an automated system for SAT practice essays, make sure students know that clarity and structure are prioritized over creative flair.
The Bottom Line
Automated essay scoring systems are powerful tools, but they're not infallible. Their accuracy and reliability depend on how they're trained, the complexity of the essays, and how you use them. By understanding their strengths and limitations, you can leverage them effectively—whether you're grading thousands of essays or helping students improve their writing skills.
The key is to use them strategically, not as a replacement for human judgment but as a complement to it. When you do, you'll save time, reduce bias, and still maintain the integrity of your assessments.
Comparing Human and Automated Essay Grading
When you compare human and automated essay grading, you're looking at two fundamentally different approaches to assessing writing quality. Human graders bring nuanced judgment and can evaluate creativity, argumentation, and subtle linguistic choices. Automated systems, on the other hand, rely on algorithms trained to identify patterns in text, focusing on quantifiable metrics like grammar, sentence structure, and word choice.
But how do they stack up against each other in practice?
Studies reveal correlations between human and automated essay scores ranging from .50 to .83, which suggests that AES systems can approximate human grading to a significant degree. However, these correlations aren't consistent across all dimensions of essay quality. For example, a study comparing IntelliMetric AES with human graders found significant alignment only in the "Sentence Structure" dimension. This tells you that while AES excels at evaluating technical aspects of writing, it struggles to capture the more subjective elements that human graders naturally assess.
Here's where it gets interesting: despite high human-rater agreement on holistic scores, automated scores sometimes show no significant correlation with human scores. This discrepancy highlights a critical limitation of AES—its inability to fully grasp the creativity, depth of argumentation, and emotional resonance that human graders can detect.
For instance, a student might craft a compelling narrative with vivid imagery, but if the essay lacks complex sentence structures or advanced vocabulary, an AES system might undervalue it.
- Key Findings:
- AES systems correlate well with human graders on technical aspects like grammar and sentence structure.
- Human graders outperform AES in evaluating creativity, argumentation, and emotional depth.
- Discrepancies often arise in holistic scoring, where AES may miss the "big picture" of an essay.
The growing use of AES in large-scale assessments, such as those by the College Board and ACT, underscores its efficiency and scalability. But as you consider relying on these systems, it's crucial to weigh their strengths against their limitations. While AES can handle high volumes of essays quickly and consistently, it may not fully capture the essence of what makes a piece of writing truly exceptional. This is why many experts advocate for a hybrid approach—using AES for initial scoring and human graders for final evaluation, especially in high-stakes scenarios.
Ultimately, the choice between human and automated grading depends on your goals. If you need speed and consistency for large-scale assessments, AES is a powerful tool. But if you're evaluating essays for creativity, depth, and originality, human graders remain indispensable. Understanding these nuances ensures you make informed decisions about how to assess writing effectively.
Applications in Large-Scale Assessments
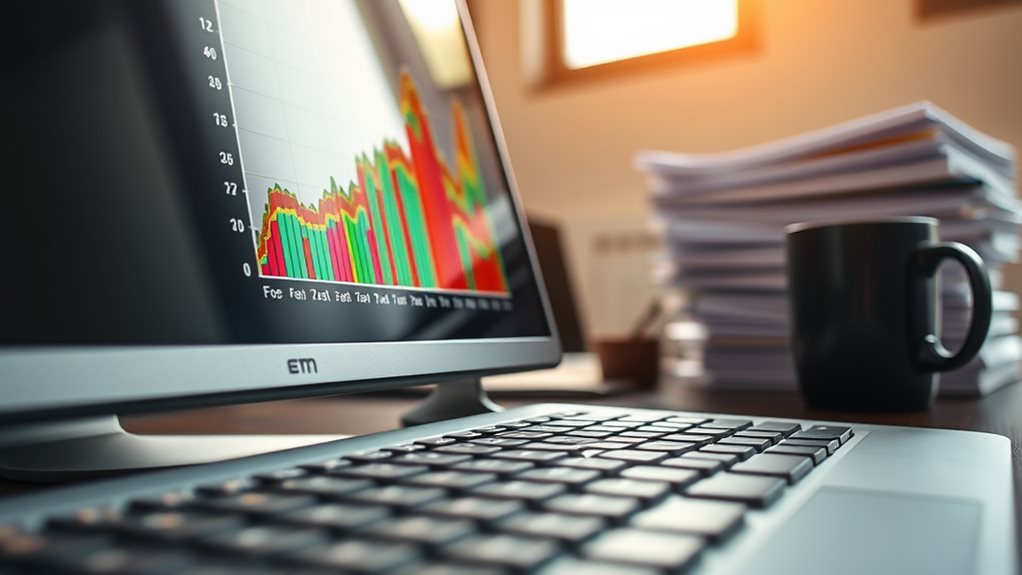
You're looking at a game-changer for large-scale assessments: automated essay scoring (AES). Imagine effortlessly grading thousands of essays in a fraction of the time it would take a human. That's the power AES brings to organizations like the College Board and ACT, where the sheer volume of essays can be overwhelming. Let's break down how it's transforming the landscape.
First, AES is all about efficiency and speed.
Picture this: instead of waiting weeks for results, students and educators get feedback almost immediately. This isn't just a convenience—it's a necessity in today's fast-paced educational environment. Systems like IntelliMetric are already proving their worth, scoring essays with a consistency that human graders can't always match.
But let's talk numbers. Take EssayGrader, for example.
It's graded over half a million essays, cutting grading time by a staggering 95%. That's not just impressive—it's revolutionary. For high-stakes assessments, where time and accuracy are critical, AES is becoming indispensable.
However, there's a caveat. While AES shows promise, its correlation with human scores varies. Studies on tests like WritePlacer Plus and THEA writing tests reveal mixed results. This means AES isn't just a plug-and-play solution—it requires ongoing validation and refinement.
Here's the bottom line: AES is cost-effective, scalable, and efficient, making it a standout choice for large-scale assessments. Yet, it's not without limitations. Nuanced aspects of writing, like creativity and depth of argument, still pose challenges. But as the technology evolves, so does its potential. You're not just adopting a tool; you're investing in the future of assessment.
Key Points:
- AES enables rapid, consistent grading for large-scale assessments.
- Systems like IntelliMetric and EssayGrader are already transforming the field.
- Immediate feedback benefits both students and educators.
- Cost-effectiveness and scalability make AES ideal for high-stakes testing.
- Ongoing validation is crucial to address limitations and improve accuracy.
You're not just navigating the present; you're shaping the future of education with AES.
Continuous Flow Scoring: A Hybrid Approach
Imagine a scoring system that adapts in real time, ensuring every response gets the attention it deserves. That's Continuous Flow Scoring—a hybrid approach that combines the speed of automation with the precision of human expertise.
Since 2015, this patented system has revolutionized large-scale assessments, scoring millions of responses with unmatched efficiency and accuracy.
Here's how it works:
- Dynamic Routing: The system instantly evaluates each response. Simple, straightforward answers are scored automatically, while complex or nuanced responses are routed to human scorers. This ensures that every answer is handled appropriately, maximizing both speed and quality.
- Real-Time Learning: The system doesn't just score—it learns. As human scorers provide feedback, the automated scoring engine refines its algorithms, improving accuracy with every interaction. This continuous improvement loop means the system gets smarter over time.
- Proven Effectiveness: Research presented at NCSA by Hauger et al. (2018) and Flanagan et al. (2016) highlights the success of this approach. It's not just a theory—it's a tested, reliable method that delivers results.
Why does this matter to you? Because it's not just about scoring faster—it's about scoring better.
By leveraging the strengths of both automation and human judgment, Continuous Flow Scoring ensures that every response is evaluated with the care and precision it deserves.
Whether you're managing a high-stakes assessment or a large-scale evaluation, this system is designed to meet your needs with unparalleled efficiency.
This isn't just a scoring system—it's a game-changer.
And it's already transforming the way assessments are handled, one response at a time.
Research and Development in Automated Scoring
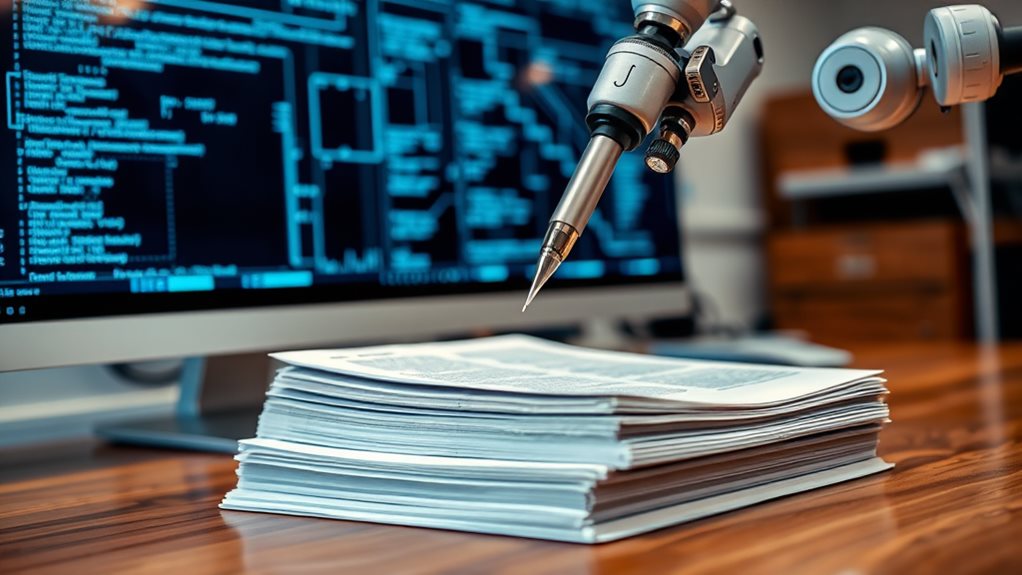
Automated essay scoring (AES) is no longer a futuristic concept—it's here, and it's evolving rapidly.
If you're in the education or assessment space, you know how critical it's to stay ahead of the curve.
The research and development behind AES is transforming how we evaluate writing, and understanding its advancements can give you a competitive edge.
The Core of AES Research
At its heart, AES relies on natural language processing (NLP) and machine learning algorithms.
These technologies analyze essays based on predefined criteria like grammar, coherence, vocabulary, and argument structure.
But here's the kicker: the latest research is pushing beyond these basics.
Developers are now integrating advanced models like GPT-4 and BERT to capture nuanced aspects of writing, such as tone, creativity, and even cultural context.
For example, imagine a student writes an essay using idiomatic expressions or regional dialects.
Traditional AES systems might flag these as errors, but cutting-edge models can now recognize and evaluate them appropriately.
This level of sophistication is a game-changer for fairness and accuracy in scoring.
Key Areas of Development
The R&D in AES is focused on solving some of the most pressing challenges in automated assessment.
Here's where the action is:
- Bias Reduction: Researchers are working tirelessly to minimize algorithmic bias. This means ensuring that essays from diverse linguistic and cultural backgrounds are scored equitably.
- Real-Time Feedback: Systems are being designed to provide instant, actionable feedback to students, helping them improve their writing on the spot.
- Adaptive Learning: AES is being integrated with adaptive learning platforms, tailoring feedback based on individual student performance over time.
- Multimodal Analysis: Beyond text, some systems are beginning to analyze visual elements like diagrams or charts in essays, broadening the scope of what can be assessed.
These advancements aren't just theoretical—they're being implemented in classrooms and testing centers worldwide.
For instance, the GRE and TOEFL exams already use AES to evaluate essays, and their systems are continuously updated to reflect the latest research.
Why This Matters to You
If you're an educator, administrator, or assessment developer, staying informed about AES research is non-negotiable.
The technology is reshaping how we think about writing assessment, and it's doing so at breakneck speed.
By leveraging these advancements, you can:
- Save time and resources while maintaining scoring accuracy.
- Provide students with more meaningful, personalized feedback.
- Ensure your assessments are fair and inclusive for all learners.
The bottom line? AES is no longer just a tool—it's a transformative force in education.
And if you're not keeping up, you're falling behind.
Dive into the research, explore the latest systems, and see how you can integrate these innovations into your work.
The future of assessment is here, and it's automated.
Ethical Considerations in Automated Essay Evaluation
When you dive into the ethical considerations of automated essay scoring (AES), you quickly realize it's not just about efficiency—it's about fairness, transparency, and the integrity of education itself. Let's break it down so you can see why this matters and how it impacts students, educators, and the future of assessment.
The Fairness Dilemma
One of the biggest ethical concerns with AES is whether it treats all students equally. Studies have shown that these systems may not perform consistently across diverse populations.
For example:
- Bias in scoring: AES algorithms might favor certain writing styles or linguistic patterns, disadvantaging students from non-native English backgrounds or those with unique cultural expressions.
- Overemphasis on surface features: If the system prioritizes grammar and mechanics over critical thinking or creativity, it risks penalizing students who excel in deeper, more nuanced aspects of writing.
This isn't just a technical issue—it's a moral one. When high-stakes assessments like college admissions or standardized tests rely on AES, you're potentially shaping futures based on flawed metrics.
The Transparency Problem
Another ethical red flag is the lack of transparency in how AES systems operate. Many of these tools are proprietary, meaning their algorithms and scoring processes are hidden from the public. This creates a trust gap.
Educators can't fully explain scores: If you're a teacher, how do you justify a student's grade when you don't understand how it was calculated?
Students feel powerless: Without insight into what the system values, they're left guessing how to improve.
This opacity undermines the educational process. If you can't see how the sausage is made, how can you trust it's fair?
The Ethical Implications of Gaming the System
Here's where things get tricky: students might adapt their writing to "beat" the AES system rather than focusing on genuine communication.
For instance:
- Formulaic writing: Students might overuse certain keywords or structures they know the system rewards, sacrificing originality and depth.
- Teaching to the test: Educators might feel pressured to train students to write for the machine, not for real-world communication.
This raises a critical question: Are we assessing writing skills, or are we assessing how well students can conform to an algorithm?
Addressing the Ethical Challenges
So, what can you do to ensure AES is used ethically? Here are some actionable steps:
- Demand transparency: Advocate for open-source AES systems or those that provide clear explanations of their scoring criteria.
- Combine human and machine scoring: Use AES as a supplementary tool, not a replacement for human graders, to balance efficiency with nuance.
- Regularly audit for bias: Test the system across diverse student populations to ensure it's fair and equitable.
By taking these steps, you can help ensure that AES serves as a tool for empowerment, not exclusion.
The Bigger Picture
At its core, the ethical debate around AES is about more than just technology—it's about the values we prioritize in education. Do we value speed and efficiency over depth and individuality? Are we preparing students for real-world communication, or just for scoring well on a machine-graded test?
As someone invested in education, you have the power to shape how AES is implemented. By pushing for fairness, transparency, and a balanced approach, you can help ensure that this technology enhances, rather than undermines, the educational experience.
The stakes are high, and the time to act is now. Let's make sure AES evolves in a way that truly serves students—not just systems.
Future Trends in Automated Essay Scoring
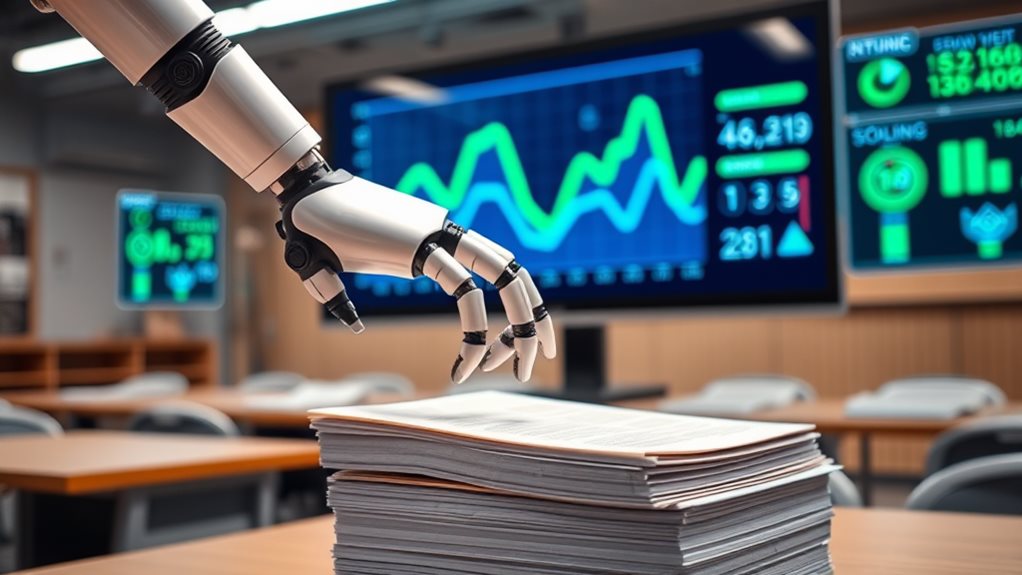
The future of automated essay scoring (AES) is poised for transformative advancements, and understanding these trends will help you stay ahead in the evolving landscape of educational technology. Let's dive into what's coming next and why it matters to you.
Nuanced Writing Evaluation
Current AES systems excel at assessing grammar, structure, and basic coherence, but they often fall short when it comes to evaluating creativity, argumentation, and stylistic nuances. Future developments will focus on bridging this gap.
Imagine a system that not only checks for grammatical accuracy but also evaluates the originality of ideas, the strength of arguments, and the emotional impact of a narrative. These advancements will make AES more holistic, ensuring it can handle the full spectrum of writing skills.
- Deep learning models will be trained on diverse datasets to recognize subtle writing qualities.
- Transformer-based architectures like GPT and BERT will enable systems to understand context and tone more effectively.
- Multimodal analysis will integrate text with other data, such as voice or visual elements, for richer evaluations.
Explainable AI (XAI) for Transparency
One of the biggest challenges with AES is the "black box" nature of its algorithms. Educators and students often struggle to trust a system when they can't understand how it arrived at a score. Future AES systems will prioritize explainability, providing clear, actionable insights into scoring decisions.
- Visual dashboards will break down scores by category (e.g., grammar, coherence, creativity).
- Real-time feedback will highlight specific areas for improvement, making the system a true teaching tool.
- Bias detection tools will ensure fairness by identifying and correcting algorithmic biases.
Integration with Adaptive Learning Platforms
AES won't operate in isolation. It will increasingly integrate with adaptive learning platforms to create a seamless, personalized assessment experience.
Picture this: a student writes an essay, the AES system evaluates it, and the adaptive platform immediately tailors the next lesson based on the student's strengths and weaknesses.
- Dynamic feedback loops will enable continuous improvement in writing skills.
- Personalized learning paths will ensure students focus on areas where they need the most help.
- Real-time analytics will give educators actionable insights to guide instruction.
Addressing Fairness and Bias
Fairness is a critical concern in AES, and future systems will prioritize techniques to mitigate bias. This includes training models on diverse datasets and implementing fairness-aware algorithms.
- Data augmentation will ensure models are exposed to a wide range of writing styles and cultural contexts.
- Fairness metrics will be embedded into the evaluation process to flag potential biases.
- Ongoing audits will keep systems accountable and transparent.
The Role of NLP and ML Breakthroughs
Advancements in natural language processing (NLP) and machine learning (ML) will be the backbone of these innovations. Transformer models, for instance, are already revolutionizing how AES systems understand and evaluate text.
- Contextual understanding will improve, allowing systems to grasp idiomatic expressions and cultural references.
- Real-time processing will make AES faster and more efficient, even for large-scale assessments.
- Multilingual capabilities will expand, enabling AES to evaluate essays in multiple languages with equal accuracy.
The future of AES isn't just about scoring essays—it's about transforming how we teach, learn, and assess writing. By staying informed about these trends, you can leverage these advancements to create more effective, equitable, and engaging educational experiences.
Best Practices for Implementing Automated Scoring Systems
When implementing automated essay scoring (AES) systems, you need to ensure the system you choose is validated with data from a population similar to your intended test-takers. This step is critical because AES systems trained on one demographic may not perform as well on another. For example, if you're assessing essays from non-native English speakers, you'll want a system that's been tested on that specific group to avoid skewed results.
Skipping this validation step could lead to inaccurate scoring, which undermines the credibility of your assessment.
To optimize efficiency and quality, consider adopting a continuous flow scoring system that combines automated and human scoring. Here's how it works: the system automatically scores straightforward essays, while routing complex or ambiguous responses to human experts. This hybrid approach ensures that nuanced or creative writing isn't penalized by rigid algorithms.
For instance, if a student uses unconventional phrasing to express a profound idea, a human scorer can recognize its value, whereas an automated system might miss it. This method not only improves accuracy but also reduces the workload on human scorers, allowing them to focus on the most challenging cases.
For large-scale assessments, AES systems like IntelliMetric, used by organizations such as the College Board and ACT, are game-changers. These systems can process thousands of essays quickly and cost-effectively, making them ideal for high-volume testing scenarios.
However, don't just rely on the system's reputation—ensure it aligns with your specific needs. For example, if you're evaluating essays for a state-wide standardized test, confirm that the system has been tested on similar datasets and meets your state's educational standards.
Establishing clear scoring rubrics is non-negotiable. You must provide transparent explanations of the evaluation methods to both students and teachers. This transparency helps avoid misinterpretations of AES results and ensures everyone understands how essays are being assessed.
For instance, if the system prioritizes grammar over creativity, students need to know this upfront so they can tailor their writing accordingly. Clear rubrics also make it easier for teachers to explain scores to students, fostering trust in the system.
Finally, consider supplementing AES with human review, especially for high-stakes assessments. Research shows that human oversight often improves accuracy by catching errors or biases that automated systems might miss.
For example, if an AES system penalizes a student for using regional slang, a human scorer can recognize it as a valid expression of the student's voice. By combining the speed of automation with the nuance of human judgment, you create a scoring process that's both efficient and fair.
Questions and Answers
Should You Fine Tune Bert for Automated Essay Scoring?
You should fine-tune BERT if accuracy gains outweigh fine-tuning costs, but consider BERT limitations like data scarcity and computational demands. Fine-tuning helps with bias mitigation, though human oversight remains essential for fairness and reliability.
What Is an Automated Scoring Engine?
An automated scoring engine uses AI to evaluate essays, focusing on scoring accuracy, bias mitigation, and time efficiency. It integrates human feedback, reduces costs, and addresses ethical concerns while streamlining assessments for learners and educators.
What Is the Essay Grading System?
An essay grading system uses a grading rubric to evaluate essays, aiming for score reliability and feedback quality. It reduces human graders' workload and bias detection issues but raises ethical concerns about fairness and transparency in assessments.