Automated essay scoring (AES) uses advanced technologies like natural language processing and machine learning to evaluate essays quickly and accurately in high-stakes exams. Systems like e-rater and Intelligent Essay Assessor analyze grammar, style, and meaning, offering faster results and cost savings. However, AES can overemphasize surface features, potentially overlooking creativity and critical thinking. Hybrid approaches combining automated and human scoring improve reliability but require careful monitoring to address biases and ensure fairness. As AES evolves, it aims to provide detailed feedback and assess higher-order skills. Exploring its potential further reveals how it shapes both testing and learning experiences.
History and Evolution of Automated Essay Scoring
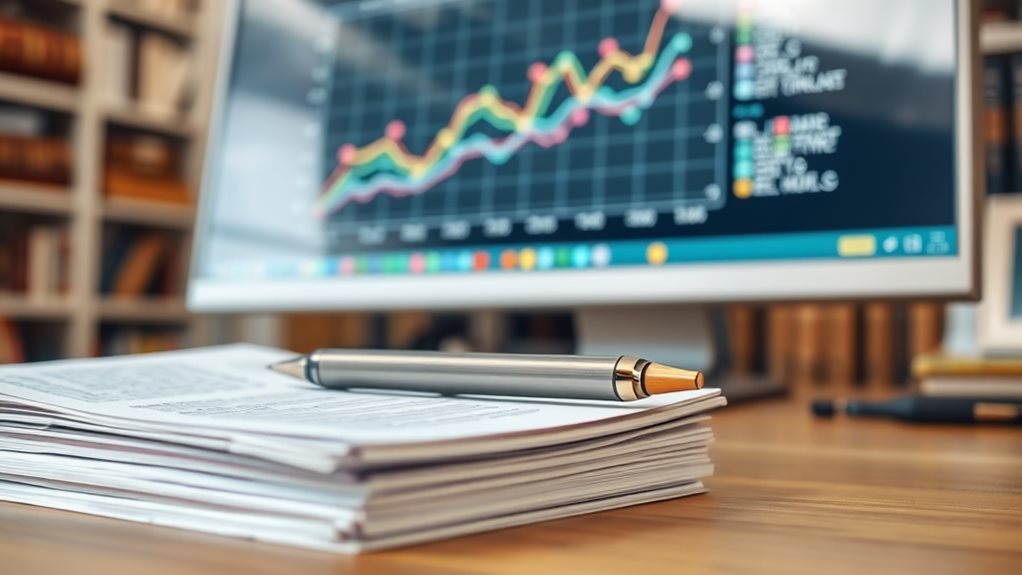
Automated essay scoring (AES) has come a long way since its inception, and understanding its history is key to appreciating how far the technology has advanced. Let's dive into the evolution of AES and explore the milestones that have shaped it into the powerful tool it is today.
In the 1960s, Project Essay Grade (PEG) emerged as one of the first attempts at automated essay scoring. Back then, the technology was limited, and the costs were prohibitive. PEG relied on surface-level features like word count and sentence length to predict essay quality.
While it was groundbreaking for its time, the lack of computational power and sophisticated algorithms meant it couldn't capture the nuances of human writing. You can imagine how frustrating it must have been for educators and researchers to see the potential but be held back by the technology of the era.
Fast forward to the 1990s, and AES experienced a resurgence. This was fueled by two major factors: the exponential growth in computing power and the development of more advanced systems like the Intelligent Essay Assessor (IEA) and e-rater. These systems introduced new techniques, such as latent semantic analysis (LSA), which allowed them to evaluate the meaning and coherence of essays rather than just surface features.
If you're familiar with machine learning, you'll recognize this as a pivotal shift—moving from simple linear regression models to more complex, data-driven approaches.
Organizations like Pearson, ETS, and Pacific Metrics began investing heavily in AES technologies, refining them over decades. For example, e-rater, developed by ETS, incorporated natural language processing (NLP) to assess grammar, usage, and even argument strength.
This was a game-changer because it meant AES systems could now evaluate not just *how* something was written but *what* was being communicated. Imagine the possibilities this opened up for standardized testing and large-scale assessments.
- Key Milestones in AES Evolution:
- 1960s: PEG introduces surface-level feature analysis.
- 1990s: IEA and e-rater leverage latent semantic analysis and NLP.
- 2000s: Machine learning techniques like deep neural networks revolutionize AES.
- Present: Proprietary algorithms dominate, but research continues to push boundaries.
Today, AES systems are more sophisticated than ever, incorporating deep neural networks and other advanced machine learning techniques. While most algorithms remain proprietary, research publications provide glimpses into how these systems have evolved. From evaluating argument strength to detecting subtle nuances in tone and style, modern AES is a far cry from its humble beginnings.
If you're working in education, testing, or even AI development, understanding this history is crucial. It's not just about knowing where AES came from—it's about seeing the trajectory and anticipating where it's headed. The evolution of AES is a testament to the power of innovation, and it's a story that continues to unfold.
Key Technologies Behind Automated Scoring Systems
Automated Essay Scoring (AES) systems rely on cutting-edge technologies to evaluate written responses with precision and efficiency. Let's break down the key technologies powering these systems so you can understand how they work and why they're so effective.
Latent Semantic Analysis (LSA)
LSA is a game-changer in text analysis. Unlike traditional keyword-based systems, LSA digs deeper to understand the *meaning* of the text. For example, Pearson's Intelligent Essay Assessor uses LSA to analyze essays by identifying semantic relationships between words and phrases. This means it can recognize that "climate change" and "global warming" are related concepts, even if the exact keywords don't appear.
It's not just about counting words—it's about understanding context and coherence.
Natural Language Processing (NLP)
NLP is the backbone of AES systems like ETS's e-rater. This technology evaluates essays on multiple dimensions:
- Grammar and usage
- Mechanics (spelling, punctuation)
- Style and tone
- Organization and development
NLP doesn't just score essays—it provides detailed feedback. For instance, if a student's essay lacks transitions between paragraphs, the system can flag this and suggest improvements.
It's like having a virtual writing coach that's available 24/7.
Machine Learning and AI
Modern AES systems leverage advanced machine learning techniques, including Bayesian inference and deep neural networks. These methods go beyond simple linear regression, allowing the system to learn from vast datasets and improve over time. For example, if thousands of essays are scored by human graders, the system can analyze these scores to refine its own scoring algorithms.
This ensures that the system becomes more accurate and reliable with each use.
Math Reasoning Engine
For open-ended math problems, Pearson's Automated Scoring Solutions use a Math Reasoning Engine. This technology evaluates not just the final answer but the entire problem-solving process. It can identify errors in logic, missing steps, or incorrect calculations, providing a comprehensive assessment of a student's mathematical reasoning.
Continuous Flow Routing
Pearson's Continuous Flow technology ensures that complex or ambiguous responses are routed to human scorers. This hybrid approach combines the speed of automation with the nuanced judgment of human experts. For example, if an essay contains unconventional phrasing or creative language that the system struggles to interpret, it's flagged for human review.
This ensures that no response falls through the cracks.
Applications of AES in High-Stakes Testing
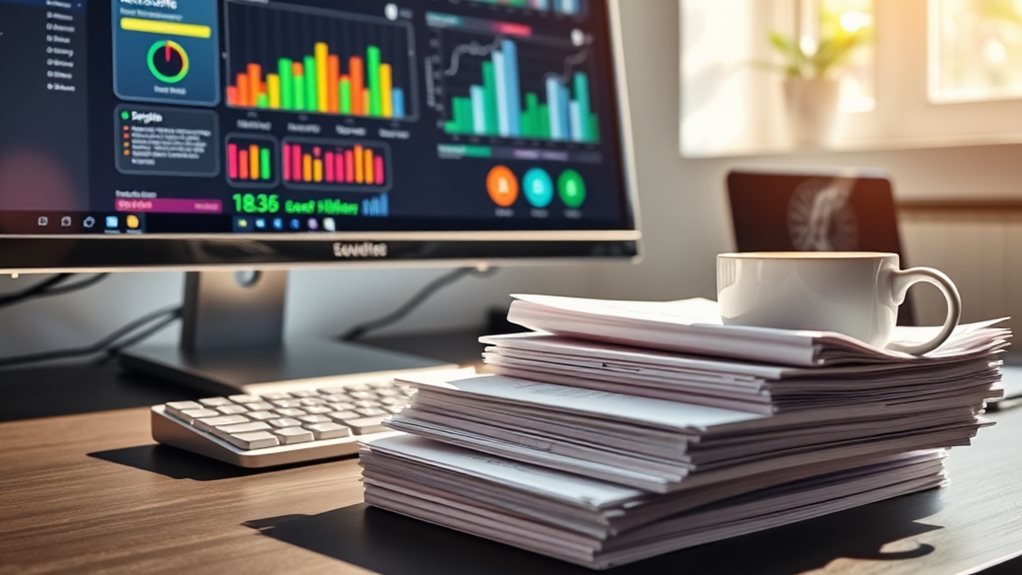
Automated Essay Scoring (AES) is revolutionizing high-stakes testing, and you need to understand how it's being applied to stay ahead. Let's dive into the real-world applications that are transforming the way assessments are scored and delivered.
First, consider the GRE and TOEFL iBT. These high-stakes exams use ETS's e-rater engine to score writing sections.
But here's the kicker: they don't rely solely on automation. Instead, they combine e-rater with human scoring to maximize reliability. This hybrid approach ensures that the nuances of language and argumentation are captured while maintaining the efficiency of automated systems. For you, this means faster results without sacrificing accuracy—a win-win for test-takers and administrators alike.
Now, let's talk about cost. High-stakes testing is expensive, especially when you factor in the frequency of these assessments. Manual grading requires significant resources, from hiring qualified graders to managing logistics.
AES offers a cost-effective alternative. By automating the scoring process, you can reduce expenses while maintaining—or even improving—the quality of assessment. This is particularly relevant for statewide writing tests, which often represent a substantial financial investment. Replacing manual grading with AES could free up resources for other critical educational initiatives.
Here's a concrete example: Pearson's Continuous Flow system. Since 2015, this system has scored millions of large-scale assessments by combining automated and human scoring. The result? Optimized efficiency and enhanced quality. For you, this means faster turnaround times and more reliable scores, which are crucial in high-stakes environments where decisions about students' futures are on the line.
But it's not just about speed and cost. Research from ETS shows that combining automated and human scoring improves the reliability and measurement benefits of assessment scores. This dual approach ensures that the strengths of both methods are leveraged, giving you the best possible outcomes. For instance, automated systems excel at consistency and scalability, while human graders bring contextual understanding and nuanced judgment. Together, they create a robust scoring system that you can trust.
Key takeaways:
- AES is being used in high-stakes exams like the GRE and TOEFL iBT, combining automation with human scoring for reliability.
- Automated scoring reduces costs, making it a viable alternative for expensive statewide writing tests.
- Systems like Pearson's Continuous Flow demonstrate the efficiency and quality benefits of combining automated and human scoring.
- Research supports the use of hybrid scoring models to enhance reliability and measurement accuracy.
Challenges and Criticisms of Automated Essay Scoring
Automated Essay Scoring (AES) has revolutionized how we assess writing, but it's not without its challenges and criticisms. If you're relying on AES for high-stakes decisions, you need to understand the limitations and potential pitfalls. Let's dive into the key issues that could impact your students, your institution, or your testing program.
Overemphasis on Surface Features
AES systems often prioritize quantifiable elements like grammar, word count, and sentence structure. While these are important, they don't capture the full picture of a student's writing ability. Creativity, originality, and critical thinking—qualities that define exceptional writing—are harder for algorithms to evaluate.
- Example: A student might write a technically flawless essay that lacks depth or originality, yet score highly because the system rewards surface-level correctness.
- Impact: This could lead to a narrow focus in teaching, where educators prioritize formulaic writing over fostering complex, nuanced skills.
Reliability Concerns
While some AES systems boast higher reliability than human raters, others struggle with consistency, especially when evaluating complex or unconventional writing.
- Studies show: Correlations between AES and human scores vary widely, with some systems achieving near-perfect alignment and others falling short.
- Takeaway: If you're using AES, you need to ensure the system aligns closely with human judgment, particularly for high-stakes assessments.
Bias and Fairness Issues
Critics argue that AES may inadvertently disadvantage marginalized student groups. Biases in the training data or algorithms can lead to unfair scoring, perpetuating inequities in education.
- Example: A system trained primarily on essays from native English speakers might struggle to accurately assess non-native speakers or students from diverse cultural backgrounds.
- Solution: Regularly audit your AES system for bias and ensure it's trained on a diverse dataset to mitigate these risks.
Cheating and AI-Generated Text
The rise of AI tools like ChatGPT has introduced new challenges for AES. Students can now generate sophisticated, human-like essays that may bypass detection systems.
- Challenge: High-stakes testing environments are particularly vulnerable to this type of cheating.
- Action Step: Combine AES with human oversight or advanced plagiarism detection tools to safeguard the integrity of your assessments.
Impact on Teaching Practices
Over-reliance on AES can lead to reductive teaching methods. Educators might focus on teaching to the test—emphasizing aspects easily scored by the system—rather than nurturing critical thinking and creativity.
- Example: Teachers might prioritize grammar drills over open-ended writing assignments, limiting students' opportunities to develop higher-order skills.
- Recommendation: Use AES as a supplementary tool, not a replacement for human evaluation, to maintain a balanced approach to writing instruction.
The Bottom Line
AES offers efficiency and scalability, but it's not a silver bullet. To use it effectively, you must address its limitations head-on. Regularly evaluate your system's performance, ensure fairness, and integrate human judgment where it matters most. By doing so, you'll strike the right balance between innovation and integrity in assessment.
Future Directions and Innovations in AES Technology
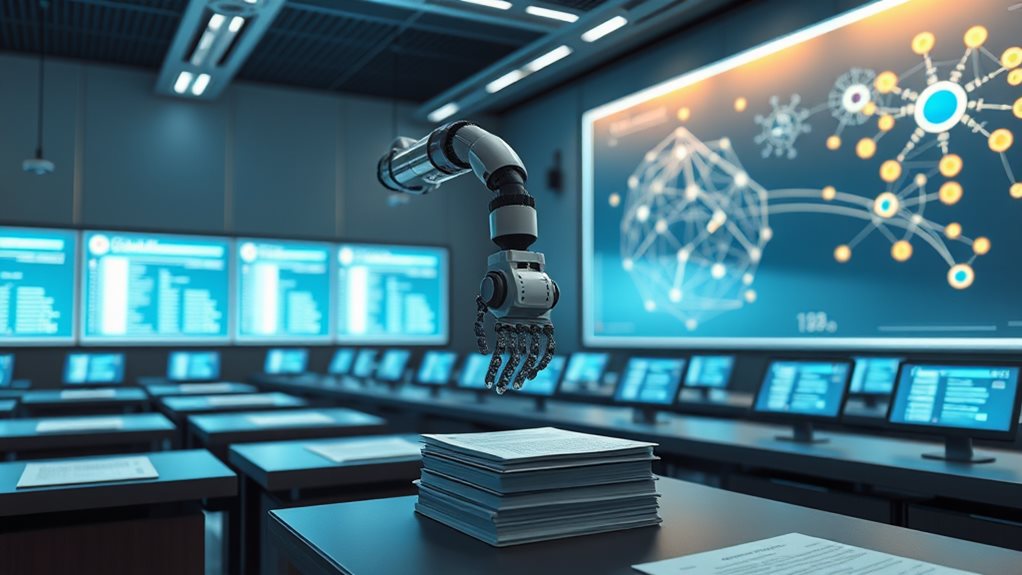
The future of Automated Essay Scoring (AES) is poised to revolutionize how we assess writing, and you'll want to stay ahead of these innovations.
As AI and machine learning continue to evolve, AES systems are becoming more sophisticated, addressing long-standing challenges like fairness and accuracy.
Imagine a system that not only evaluates grammar and mechanics but also understands the nuances of argumentation, creativity, and even cultural context.
This isn't just a pipe dream—it's happening now, and it's reshaping the landscape of education.
Here's what you can expect in the near future:
- AI-Driven Fairness and Accuracy: Researchers are leveraging AI to reduce bias in AES systems. By training algorithms on diverse datasets, these systems can better handle variations in writing styles, dialects, and cultural expressions. This means more equitable scoring for all students, regardless of their background.
- Nuanced Feedback: Future AES tools won't just spit out a score. They'll provide detailed, actionable feedback. Think of a system that identifies weak arguments, suggests stronger vocabulary, or even highlights areas where creativity could be enhanced. This level of insight will empower students to improve their writing in meaningful ways.
- Expanded Competency Assessment: Beyond grammar and mechanics, AES is moving toward evaluating higher-order skills. Can a student construct a compelling argument? Are they using evidence effectively? These are the kinds of questions AES will soon answer, giving educators a more holistic view of student writing.
- Real-Time Integration: Imagine a classroom where students receive instant feedback as they write. Future AES systems will integrate seamlessly with instructional tools, offering real-time suggestions and personalized learning pathways. This isn't just about grading—it's about fostering growth.
But here's the kicker: these advancements aren't just about technology. They're about transforming teaching and learning.
As AES becomes more sophisticated, it will free up educators to focus on what they do best—mentoring and guiding students.
At the same time, students will gain access to tools that help them refine their skills in ways that were previously unimaginable.
The impact of these innovations is already being felt, and the pace of change is accelerating.
If you're an educator, administrator, or even a student, now is the time to embrace these advancements.
The future of AES isn't just about scoring essays—it's about unlocking potential.
And that's a future worth investing in.
Questions and Answers
How Does Automated Essay Scoring Work?
You'll see automated essay scoring use a scoring rubric to evaluate essays. It performs feature extraction, human comparison, and bias detection while analyzing natural language. It also considers contextual understanding, error analysis, model limitations, ethical concerns, and future prospects.
Should You Fine Tune Bert for Automated Essay Scoring?
You should fine-tune BERT for automated essay scoring if you've got quality data and resources to address fine-tuning costs, BERT limitations, and data scarcity. Prioritize bias mitigation, human oversight, and score reliability to ensure ethical, practical applications.
What Is an Automated Scoring Engine?
An automated scoring engine uses algorithms to evaluate essays, balancing engine types, scoring biases, and system limitations. You'll consider cost factors, ethical concerns, data security, and legal aspects while ensuring human oversight and vendor selection aligns with future trends.
What Is the Analytic Method of Scoring Essay Test?
You'll use an analytic rubric to break essay quality into traits like content analysis, grammar assessment, and style analysis. It's more detailed than holistic scoring, offering feedback mechanisms and improving scoring reliability through error detection.