Automated Essay Scoring (AES) systems analyze essays for grammar, structure, and content, providing insights with accuracy rivaling human graders (correlations in the mid-.80s). You can use AES data to identify common errors like vocabulary misuse or poor organization, enabling targeted interventions. For example, algorithms like PEG improve writing quality by 22%. AES analytics categorize feedback into areas like Development of Ideas, helping you adjust instruction efficiently. By pinpointing class-wide weaknesses, you can allocate resources effectively and track student progress. These tools also free up time for personalized support. Exploring further will reveal strategies to maximize AES for impactful teaching outcomes.
Understanding Automated Essay Scoring Systems
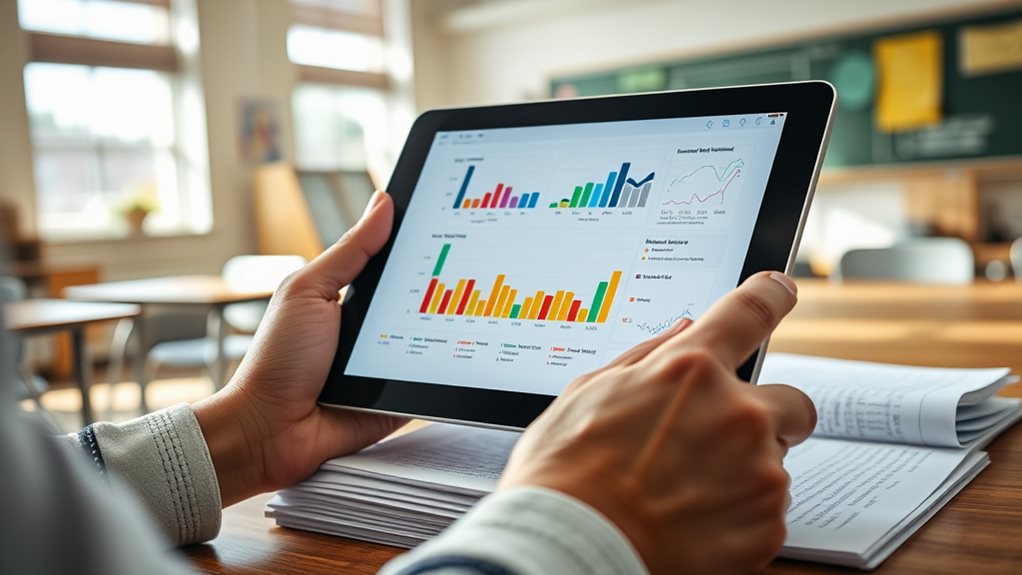
Automated Essay Scoring (AES) systems are revolutionizing how writing is assessed, and understanding how they work is key to leveraging their potential. These systems, like PEG, don't just spit out a score—they analyze a wide range of writing characteristics to predict how a human rater would evaluate an essay.
Think of it as a highly sophisticated tool that breaks down your writing into measurable components, from sentence fluency and vocabulary usage to content relevance and coherence. The result? Predictive accuracy that often rivals human scoring, with correlations in the mid-.80s.
But here's what you need to know: AES systems aren't just about scoring. They're about understanding the nuances of writing.
For instance, PEG evaluates specific traits like Development of Ideas, Organization, and Style, giving you actionable insights into where your writing shines—and where it needs work. Other systems might focus on holistic scoring, but the best ones dig deep, using a mix of statistical, style-based, and content-based features to assess essays.
- Key Features Assessed by AES Systems:
- Sentence fluency and grammatical accuracy
- Vocabulary diversity and sophistication
- Content relevance and depth
- Organization and coherence
- Style and tone consistency
The accuracy of these systems is often measured using metrics like Quadratic Weighted Kappa (QWK), with some studies achieving scores above 0.887. That's not just impressive—it's a game-changer for educators and students alike.
But here's the kicker: AES systems can also replicate human biases. If human raters tend to favor certain writing styles or penalize others, AES systems might pick up on those patterns. That's why it's crucial to understand how these systems are trained and what data they're built on.
When you're working with AES, you're not just getting a score—you're getting a roadmap for improvement. Whether you're a teacher looking to provide targeted feedback or a student aiming to refine your writing, these systems offer a level of detail and precision that's hard to match.
And with machine learning techniques like Ridge regression and neural networks driving the analysis, the insights you get are both data-driven and actionable.
Key Metrics for Evaluating AES Performance
When evaluating Automated Essay Scoring (AES) systems, you need to focus on key metrics that truly measure their effectiveness. These metrics not only tell you how well the system performs but also highlight areas for improvement. Let's break them down so you can understand their significance and how they impact your decision-making.
Quadratic Weighted Kappa (QWK) is the gold standard for AES evaluation. It doesn't just measure agreement between automated and human scores—it accounts for the severity of disagreements. For example, if the system gives a score that's two points off from the human rater, QWK penalizes that more heavily than a one-point discrepancy. This makes it a robust metric for assessing score-level agreement.
In fact, recent studies show that adding auxiliary tasks like prompt prediction and prompt matching can boost QWK by 2.5% on average. That's a significant improvement in a field where even small gains matter.
Pearson Correlation Coefficient (PCC) is another critical metric. It measures the linear relationship between automated and human scores, giving you insight into how closely the system's predictions align with human judgment. A PCC in the mid-.80s is considered strong, indicating that the AES system is capturing the nuances of human scoring effectively.
However, PCC alone isn't enough—it doesn't account for systematic biases or large errors, which is why you need to pair it with other metrics.
Mean Absolute Error (MAE) and Mean Squared Error (MSE) are your go-to metrics for understanding the magnitude of errors. MAE gives you the average difference between automated and human scores, making it easy to interpret. MSE, on the other hand, squares the errors, giving more weight to larger discrepancies. This is particularly useful when you want to identify and address outliers that could skew your results.
For instance, if your AES system occasionally produces wildly inaccurate scores, MSE will highlight those instances, prompting you to investigate and refine the model.
- QWK: Measures agreement with severity weighting.
- PCC: Assesses linear relationship strength.
- MAE: Provides average error magnitude.
- MSE: Highlights large errors for targeted improvements.
Identifying Common Writing Errors With AES
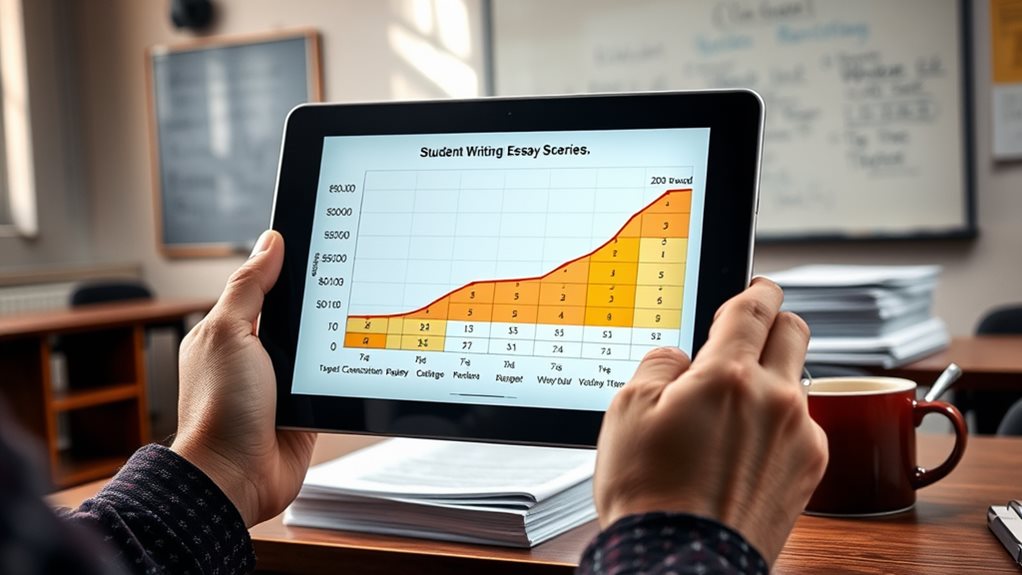
Automated Essay Scoring (AES) systems are revolutionizing how you identify and address common writing errors in your students. These tools don't just grade essays—they provide a detailed breakdown of where students struggle most, giving you actionable insights to improve instruction.
Let's dive into how AES can help you pinpoint those persistent issues.
First, consider the power of algorithms like PEG. Studies show that students who receive feedback from PEG improve their writing quality by 22%.
Why? Because AES systems analyze every aspect of writing—sentence fluency, word choice, grammar, and more. They don't just tell students they made a mistake; they highlight exactly what went wrong and often suggest how to fix it. For example, if a student consistently misuses commas in compound sentences, the system flags it, allowing you to target that specific skill in your lessons.
Here's what AES can uncover for you:
- Grammatical errors: From subject-verb agreement to misplaced modifiers, AES identifies patterns of mistakes that might slip past even the most attentive teacher.
- Sentence structure issues: Fragments, run-ons, and awkward phrasing are flagged, helping students refine their syntax.
- Vocabulary misuse: Overused words, vague language, or incorrect word choices are highlighted, pushing students toward more precise expression.
- Organization problems: AES can detect when essays lack coherence or fail to follow a logical structure, guiding students to improve their flow.
But it's not just about identifying errors—it's about understanding trends.
By analyzing large datasets, like the HSK Dynamic Composition Corpus, AES systems reveal common pitfalls across thousands of essays. For instance, if 60% of your students struggle with transitions between paragraphs, you know exactly where to focus your next lesson. This data-driven approach ensures your instruction is targeted and effective.
What's more, AES systems are incredibly accurate, often exceeding the reliability of human graders. With inter-rater reliability scores in the mid-.80s, these tools consistently pinpoint errors that even experienced teachers might miss. And because they replicate the biases present in human scoring, they offer a realistic picture of where students need the most help.
Tailoring Instruction Based on AES Insights
Automated Essay Scoring (AES) data isn't just about grading essays faster—it's a goldmine for tailoring instruction to meet your students' unique needs. By leveraging insights from AES systems like PEG or MI Write, you can pinpoint exactly where your students struggle and craft targeted interventions that drive real improvement. Let's break down how you can use these insights to transform your teaching approach.
Identify Common Weaknesses Across Your Class
AES systems provide detailed analytics on student performance, categorizing feedback into areas like Development of Ideas, Organization, Style, Word Choice, Sentence Fluency, and Conventions.
For example, if PEG highlights that 60% of your class struggles with Organization, you can design a mini-lesson series focused on structuring essays effectively. This targeted approach ensures you're addressing the most pressing needs, saving you time and maximizing impact.
- Actionable Insight: Use AES data to identify patterns in student errors.
- Example: If Word Choice is a recurring issue, introduce vocabulary-building exercises or mentor texts that model strong word selection.
Personalize Feedback for Individual Growth
One of the most powerful features of AES is its ability to provide immediate, individualized feedback.
Imagine a student receives a low score in Sentence Fluency. Instead of waiting for your next writing conference, they can revise their work right away, using the AES feedback as a guide. This iterative process accelerates learning and empowers students to take ownership of their progress.
- Actionable Insight: Encourage students to use AES feedback for self-directed revision.
- Example: Pair AES feedback with peer review sessions, where students discuss how they addressed specific issues like Style or Conventions.
Leverage Multi-Task Learning Models for Nuanced Adjustments
Advanced AES models, like the NEZHA encoder with auxiliary tasks, offer even deeper insights. These systems analyze not just the essay but also how well it matches the prompt and aligns with the expected response.
If your students consistently score low on prompt matching, it's a sign they need more practice understanding and addressing writing prompts effectively.
- Actionable Insight: Use AES data to identify gaps in prompt comprehension.
- Example: Create activities where students deconstruct prompts and brainstorm how to align their essays with the requirements.
Bridge the Gap for ELL Students
Research shows that AES systems like MI Write are just as effective as human scoring for English Language Learners (ELLs). This means you can confidently use AES data to tailor instruction for ELL students, focusing on areas like Conventions or Sentence Fluency where they may need extra support.
- Actionable Insight: Use AES feedback to design scaffolded writing tasks for ELL students.
- Example: Provide sentence frames or graphic organizers to help ELL students improve Organization and Development of Ideas.
Save Time While Enhancing Instruction
With AES handling the initial scoring and feedback, you're freed up to focus on higher-level instructional strategies. Instead of spending hours grading, you can analyze AES data to identify trends, plan targeted lessons, and provide one-on-one support where it's needed most.
- Actionable Insight: Use AES analytics to streamline your lesson planning.
- Example: If AES data shows a class-wide struggle with Conventions, dedicate a week to grammar and punctuation mini-lessons.
Integrating AES Data Into Classroom Practices
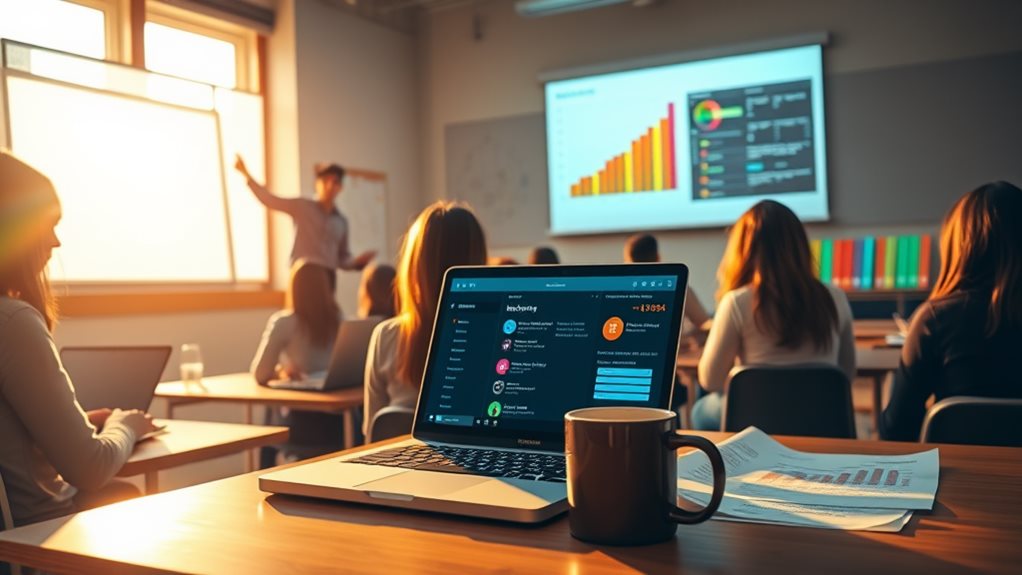
Automated essay scoring (AES) data isn't just a tool for grading—it's a game-changer for classroom instruction. When you integrate AES data into your teaching practices, you unlock a wealth of insights that can transform how you support your students. Let's break down how you can leverage this data to elevate your instruction and drive student growth.
Pinpoint Student Needs with Precision
AES systems like PEG provide detailed analytics on student performance, giving you objective data to identify exactly where your students are struggling. For example, if PEG highlights consistent issues with sentence structure or vocabulary usage across your class, you can tailor your lessons to address these specific areas. This targeted approach ensures you're not wasting time on skills your students have already mastered.
- Identify struggling learners early: AES data can flag students who are falling behind, allowing you to intervene before gaps widen.
- Track progress over time: Use longitudinal data to measure growth and adjust your teaching strategies accordingly.
- Focus on high-impact areas: Prioritize instruction on the skills that will make the biggest difference in student outcomes.
Save Time While Enhancing Feedback
One of the most significant benefits of AES is the time it saves you. Instead of spending hours grading essays, you can use PEG's automated feedback to provide students with immediate, actionable insights. This frees up your time to focus on what really matters—delivering high-quality instruction and personalized support.
For instance, ERB Writing Practice, powered by PEG, offers over 500 prompts and lessons, giving you a ready-made resource to integrate into your curriculum. You can use the data from these exercises to identify trends and adjust your teaching in real time.
Eliminate Bias and Ensure Fairness
AES systems like PEG remove the subjectivity often associated with essay scoring. By relying on objective data, you can ensure that every student is evaluated fairly, regardless of personal biases. This is especially valuable when assessing a diverse classroom, as it allows you to identify strengths and weaknesses across your entire student population without prejudice.
Drive Immediate Action with Real-Time Insights
The real-time feedback provided by AES systems is a game-changer for classroom instruction. When students receive instant feedback on their writing, they can make adjustments right away, reinforcing learning while it's still fresh. For you, this means you can monitor progress in real time and pivot your teaching strategies as needed.
- Adjust lessons on the fly: Use real-time data to tweak your instruction mid-unit.
- Personalize interventions: Identify students who need extra help and provide targeted support.
- Celebrate progress: Use data to highlight student growth and build confidence.
Build a Data-Driven Classroom Culture
Integrating AES data into your classroom isn't just about improving instruction—it's about fostering a culture of data-driven decision-making. When students see how their performance data informs your teaching, they become more invested in their own growth. Share insights from PEG with your students, and use the data to set individualized goals and track progress together.
Addressing Challenges in AES Implementation
Automated Essay Scoring (AES) systems promise efficiency and scalability, but their implementation isn't without challenges. If you're considering adopting AES, you need to be aware of the hurdles and how to navigate them effectively. Let's break down the key issues and solutions to ensure you're making the most of this technology.
Bias in Scoring: A Persistent Challenge
One of the most pressing concerns with AES is its tendency to replicate human biases. A study of 2,829 elementary students revealed that AES systems, like MI Write, mirrored the same biases found in human scoring. This means essays from certain demographics—such as English Language Learners (ELLs)—may be unfairly assessed.
- Why it matters: If your AES system isn't trained on diverse datasets, it risks perpetuating inequities in assessment.
- What you can do: Advocate for AES systems that include robust, representative training data. Push for transparency in how these systems are developed and tested for bias.
Lack of Representation in Training Data
Research shows that many AES systems lack sufficient representation of ELLs in their training data. This omission can lead to predictive bias, where the system struggles to accurately score essays from these students.
- Why it matters: If your student population includes ELLs, an AES system that doesn't account for their unique linguistic patterns could undermine their academic progress.
- What you can do: Work with vendors to ensure their systems are trained on diverse datasets. Consider supplementing AES with human grading for ELLs to ensure fairness.
Accuracy Variability Across Models
The accuracy of AES systems isn't universal—it varies depending on the model and dataset used. For example, one study achieved 0.887 accuracy using a ridge regression model, while others reported lower accuracies (e.g., 0.532, 0.77).
- Why it matters: If you're relying on AES for high-stakes assessments, inconsistent accuracy can lead to unreliable results.
- What you can do: Evaluate multiple AES systems and choose one with proven accuracy for your specific use case. Look for models that incorporate advanced features, like prompt prediction and matching, which have shown improvements in performance metrics like QWK and PCC.
Balancing Automation with Human Insight
While AES systems excel at handling large volumes of essays, they often lack the nuanced understanding of essay meaning that human graders provide. This can result in feedback that feels generic or misses the mark.
- Why it matters: Students need meaningful feedback to grow as writers, and overly automated systems may fall short.
- What you can do: Use AES as a tool to complement, not replace, human grading. Pair automated scoring with detailed, personalized feedback from educators to create a more holistic assessment process.
The Path Forward: Addressing Challenges Head-On
Implementing AES isn't just about adopting new technology—it's about ensuring that technology serves your students and educators effectively. By addressing biases, demanding diverse training data, and balancing automation with human insight, you can harness the power of AES to improve instruction and assessment outcomes.
- Key takeaways:
- Push for transparency and diversity in AES training data.
- Evaluate AES systems for accuracy and suitability to your needs.
- Combine automated scoring with human feedback for a balanced approach.
The challenges of AES implementation are real, but with the right strategies, you can overcome them and unlock the full potential of this powerful tool.
Future Trends in AES and Education
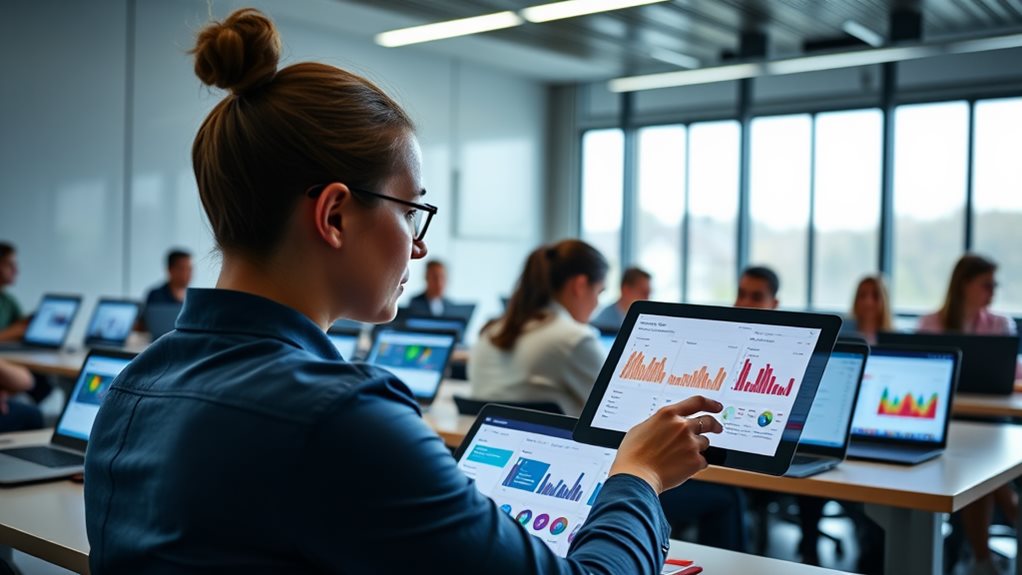
The future of Automated Essay Scoring (AES) is poised to revolutionize education, and you need to be ready for what's coming. As an educator or stakeholder, understanding these trends will help you stay ahead and leverage these advancements to their fullest potential. Let's dive into the key developments shaping the future of AES and how they'll impact teaching and learning.
Multimodal Prompt Data Integration
One of the most exciting trends is the move toward incorporating multimodal data into AES systems. Imagine an AES tool that doesn't just analyze text but also evaluates visual or auditory prompts. For example, if students are asked to write an essay based on a video or an infographic, future AES systems will be able to assess how well their writing aligns with the multimodal source material. This approach will:
- Provide a more holistic evaluation of student comprehension and critical thinking.
- Enable personalized feedback based on how students interpret and synthesize information from diverse formats.
- Prepare students for real-world tasks that require integrating multiple types of information.
Dynamic Parameter Optimization
Another game-changer is the use of dynamic parameter optimization in AES systems. Instead of relying on static models, these systems will adapt their scoring parameters based on the specific context of the assignment or the individual student's learning trajectory. For instance:
- If a student consistently struggles with coherence, the system could adjust its feedback to focus more on that area.
- For advanced writers, the system might prioritize nuanced aspects like argument depth or stylistic sophistication.
- This adaptability ensures that AES tools remain relevant and effective across diverse educational settings and student needs.
Addressing Bias and Fairness
As AES systems become more sophisticated, addressing bias—particularly against English Language Learners (ELLs)—remains a critical focus. Future advancements will prioritize fairness by:
- Training models on more diverse datasets to reduce cultural and linguistic biases.
- Incorporating explainable AI to ensure transparency in how scores are determined.
- Providing tailored feedback that accounts for language proficiency levels, helping ELLs improve without penalizing them unfairly.
The Role of AI in Personalized Learning
AES is evolving beyond just scoring essays—it's becoming a tool for personalized learning. With AI-driven insights, you'll be able to:
- Identify specific areas where each student needs improvement, from grammar to argument structure.
- Offer targeted resources, like practice exercises or instructional videos, based on individual performance.
- Track progress over time, giving you a clear picture of how students are developing their writing skills.
Preparing for the Future
To stay ahead, you'll want to:
- Familiarize yourself with the latest AES tools and their capabilities.
- Advocate for the integration of these technologies in your institution.
- Encourage professional development for educators to effectively use AES systems in the classroom.
The future of AES isn't just about automating grading—it's about transforming how we teach, learn, and assess writing. By embracing these trends, you'll be better equipped to support your students and prepare them for the demands of the 21st century.
Questions and Answers
How Does Automated Essay Scoring Work?
Automated essay scoring uses scoring algorithms to analyze essays. You'll see feature engineering extract writing traits, data preprocessing clean inputs, and model evaluation ensure accuracy. It addresses bias mitigation, human feedback, ethical concerns, system limitations, future directions, and practical applications.
Can Automated Writing Evaluation Programs Help Students Improve Their English Writing?
You can use automated writing evaluation programs to improve English writing by addressing writing anxiety, tailoring feedback to learning styles, and tracking progress. However, system limitations and ethical concerns require teacher training and thoughtful assignment design.
Should You Fine Tune Bert for Automated Essay Scoring?
You should fine-tune BERT for automated essay scoring if you've addressed BERT limitations, conducted a cost-benefit analysis, and ensured sufficient data requirements. Consider transfer learning, model bias, human oversight, ethical concerns, interpretability issues, real-world impact, and generalizability challenges.
What Is the AES Scoring System?
An AES scoring system evaluates essays using algorithms, assessing traits like grammar and coherence. You'll consider AES reliability, validity, bias, fairness, ethics, cost, access, impact, future, and limitations to ensure it supports equitable, effective, and scalable writing instruction.