Automated essay grading raises ethical concerns around fairness, privacy, and transparency. Algorithms can exhibit bias, especially against non-native speakers or diverse linguistic groups, due to skewed training data. Student data privacy is also at risk, as sensitive information must be securely handled to prevent misuse. The "black box" nature of AI makes it hard to explain grading decisions, undermining trust. While automation saves time, it risks reducing personalized feedback and weakening student-instructor relationships. Balancing efficiency with ethical practices requires diverse datasets, robust privacy measures, and human oversight. Exploring these challenges further reveals how to responsibly integrate AI in education.
Algorithmic Bias
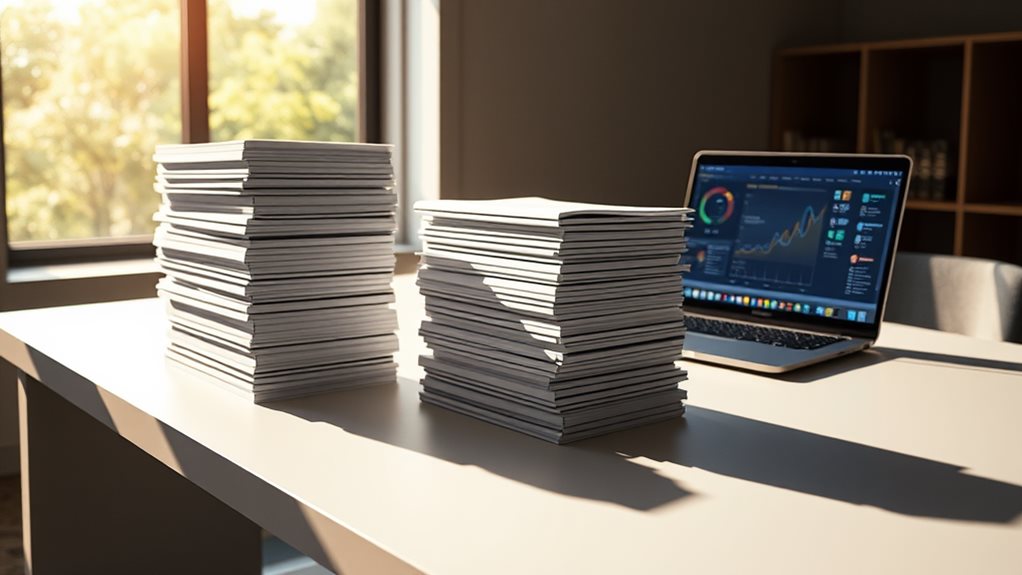
When you implement automated essay scoring (AES) systems, you must confront the reality of algorithmic bias—a challenge that can undermine fairness in education. These biases often stem from the training data used to develop the AI or the design of the algorithms themselves.
For instance, if the training data disproportionately represents one demographic or linguistic group, the system may disadvantage others. This isn't just theoretical; it's a practical issue that can affect real students.
Consider this example: a study using a textbook vendor's AI software to grade business law essays revealed troubling discrepancies in scoring. The software struggled to align with specific linguistic and cultural nuances, raising questions about its accuracy and fairness for diverse student populations.
If you're relying on these tools, you need to ask: does this software truly understand the context of the essays it's grading, or is it missing the mark?
Native-language bias is another critical concern. Students whose first language isn't the language of instruction often face unfair grading outcomes because AES systems may penalize non-native phrasing or structures.
In the Malaysian University English Test (MUET) context, for instance, using commercial AES software could lead to scores that don't accurately reflect Malaysian standards or student abilities. Are you confident that your chosen software avoids this pitfall?
To address algorithmic bias effectively, you need to take proactive steps:
- Choose AI platforms carefully: Evaluate whether the software has been trained on diverse data sets that reflect your student population.
- Implement bias prevention strategies: Regularly audit the system's performance across different student groups to identify and correct disparities.
- Assess fairness and accuracy: Continuously monitor AI feedback to ensure it aligns with human grading standards and doesn't perpetuate inequities.
Data Privacy Risks
When you implement automated essay grading systems, you're not just dealing with algorithms—you're handling sensitive student data. This raises significant privacy risks that you can't afford to overlook.
Systems often store student writing samples, including personal reflections, creative work, and academic essays, which, if compromised, could lead to serious breaches of trust and regulatory violations.
Consider this: third-party vendors frequently process this data, and without stringent safeguards, unauthorized access or misuse becomes a real threat. The algorithms themselves may retain student information, creating vulnerabilities that hackers or even internal employees could exploit.
If you're not transparent about how this data is stored and who's access, you risk violating privacy laws like FERPA or GDPR.
Here's what you need to watch out for:
- Data retention policies: Many systems don't clearly state how long student work is stored or when it's deleted. This lack of clarity can lead to unintended data exposure.
- Third-party risks: When student data is transferred to vendors for processing, it's often outside your direct control. Ensure they comply with privacy regulations and use encryption.
- Algorithmic transparency: If the system's inner workings are a "black box," you mightn't even know what data is being retained or shared.
- Unauthorized access: Weak security measures can expose student essays to hackers, leading to potential misuse or public disclosure.
You must take proactive steps to mitigate these risks. Implement end-to-end encryption, ensure vendors follow strict data protection protocols, and educate stakeholders about the importance of safeguarding student work. The urgency here is real—every day without strong privacy measures in place increases the likelihood of a breach that could damage your institution's reputation and harm students.
Transparency Challenges
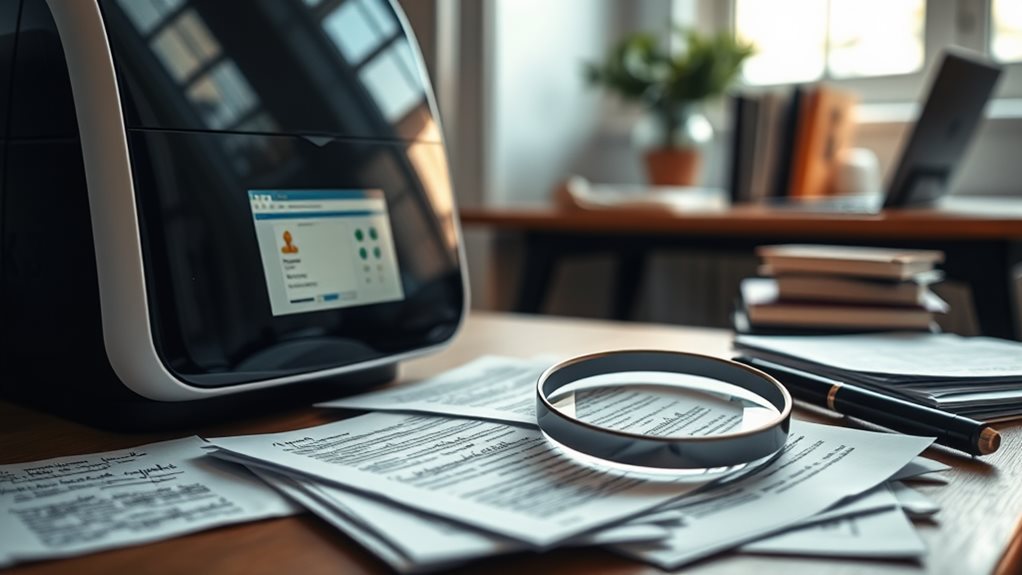
When implementing automated essay grading systems, transparency isn't just a bonus—it's a necessity. You're dealing with students, educators, and administrators who rely on these tools to make critical decisions about learning outcomes. If they can't understand how the system works, trust erodes, and the technology loses its value.
One of the biggest challenges? *Explaining the "black box" nature of AI algorithms.* Most automated grading systems rely on complex machine learning models that analyze text for grammar, coherence, and relevance. But how does the system decide a score?
If you can't clearly articulate the criteria, stakeholders will question its fairness and accuracy.
- Lack of explainability: Without clear insights into how scores are generated, educators may feel sidelined, and students may feel unjustly assessed.
- Bias detection: If the system's decision-making process isn't transparent, identifying and mitigating biases becomes nearly impossible.
- User confidence: When educators and students don't understand the grading logic, they're less likely to trust the results, undermining the system's effectiveness.
To address these challenges, you need to prioritize explainability. Consider integrating tools that provide detailed feedback on why a score was assigned. For example, highlighting specific areas of improvement (e.g., sentence structure, argument clarity) can make the process more transparent and actionable.
Additionally, involve educators in the development and testing phases. Their input ensures the system aligns with teaching goals and remains interpretable to end-users. Transparency isn't just about revealing the mechanics—it's about fostering trust and ensuring the technology serves its intended purpose.
Without transparency, your grading system risks becoming a liability rather than an asset. Address these challenges head-on, and you'll create a tool that educators and students can rely on with confidence.
Student-Instructor Impact
When you introduce AI grading systems into your classroom, you're not just changing how essays are evaluated—you're reshaping the dynamic between you and your students. This shift isn't just administrative; it's deeply relational.
Think about it: when you rely on algorithms to assess student work, you risk losing the personalized feedback that builds trust and fosters growth. Your students might start tailoring their essays to please the machine rather than engaging with you, their instructor. And that's where the real problem lies.
Here's what you need to consider about the student-instructor impact:
- Loss of Personalized Feedback: AI can analyze grammar or structure, but it can't replicate the nuanced, motivational feedback you provide. When your feedback is automated, students miss out on the mentoring that helps them grow.
- Erosion of Rapport: The student-instructor relationship thrives on interaction. If AI handles grading, that human connection weakens, and students may feel less supported in their learning journey.
- Algorithm-Driven Writing: Students might start optimizing their essays for the AI's criteria rather than focusing on critical thinking or creativity. This shifts the focus from learning to compliance, which undermines the purpose of education.
Imagine a student struggling with a complex legal concept in their business law essay. Instead of sitting down with you to discuss their approach, they're left with a generic AI comment like, "Improve clarity." That doesn't help them understand where they went wrong or how to improve. It's feedback without direction, and it leaves them feeling disconnected.
And let's not forget the urgency here. The longer you rely on AI grading, the more this dynamic solidifies. Students might begin to see you as less involved in their learning process.
Over time, this could erode their motivation and engagement.
So, what's the solution? Use AI as a tool, not a replacement. Let it handle the repetitive tasks—like grammar checks or basic formatting—so you can focus on providing the thoughtful, personalized feedback that makes a difference. This way, you preserve the student-instructor relationship while still leveraging technology to save time.
The bottom line? AI has its place, but it shouldn't come at the expense of the human connection that drives meaningful learning. Keep the focus on mentoring, and remember: your role as an instructor is irreplaceable.
Fairness and Accuracy
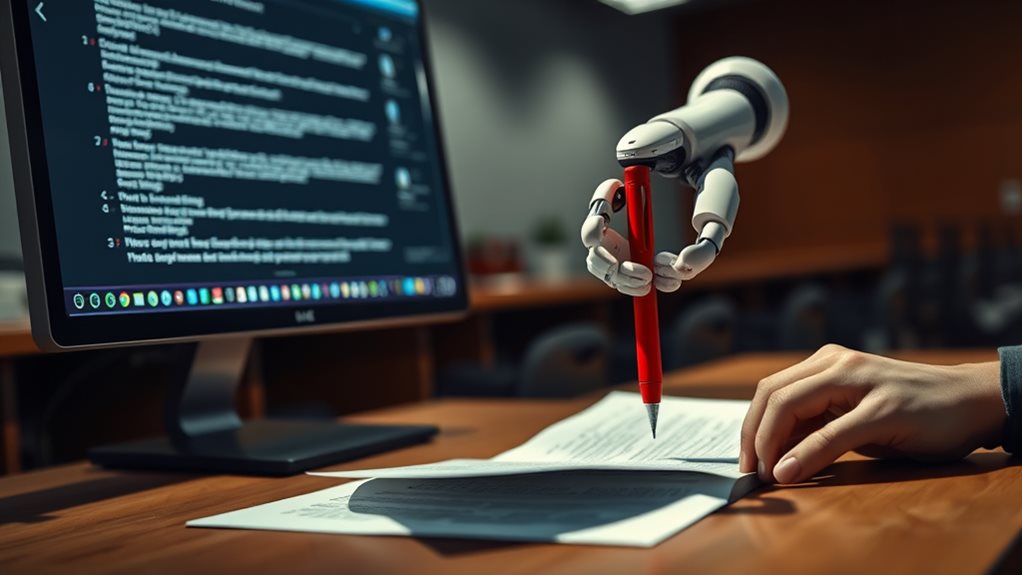
Let's dive into the fairness and accuracy of automated essay grading—because this isn't just about efficiency; it's about equity.
You're dealing with a system that claims 100% reliability, but as someone who's worked in this space, I can tell you it's not that simple.
Algorithmic bias is real, and it disproportionately impacts specific groups, like second language learners.
Imagine a student who's worked tirelessly on their business law essay, only to be graded lower because the AES software wasn't trained on data that reflects their linguistic background.
That's not just unfair; it's damaging to their academic journey.
AES systems rely heavily on their training datasets, and if those datasets are skewed toward native-language speakers, the bias becomes systemic.
In a pilot study involving 27 students, concerns were raised about whether AES could accurately grade essays from diverse linguistic and cultural contexts.
Take the Malaysian University English Test (MUET), for example.
If the AES isn't tailored to the specific nuances of Malaysian English, it risks misjudging students' work.
Now, let's talk accuracy.
The claim of 100% reliability comes from the consistency of algorithmic grading—no fatigue, no mood swings, no deviations.
But essays are inherently subjective.
They're not math problems with clear right or wrong answers.
Automated systems might miss subtle arguments, creative phrasing, or cultural references that a human grader would recognize.
This isn't to say AES has no place in grading; it's a tool that can be incredibly useful.
But you need to approach it with a critical eye.
- Bias in training data: Algorithms reflect the data they're trained on. If the dataset lacks diversity, the grading will be biased.
- Cultural context: Essays often reflect cultural nuances. AES systems must be adaptable to these variations.
- Subjectivity of essays: Unlike multiple-choice tests, essays require judgment that algorithms may not handle well.
Here's the kicker: manual grading, while time-consuming, remains the gold standard for comparison.
Yes, human graders can be inconsistent, but they also bring a level of understanding and flexibility that algorithms currently can't match.
When you're evaluating the fairness and accuracy of AES, inconsistencies in human grading can actually serve as a benchmark.
It's not about perfection—it's about alignment with human judgment.
Human Oversight Needs
You can't fully trust automated essay grading systems to handle the complexities of human language and cultural nuances on their own. Even with advanced AI, these systems still require human oversight to ensure fairness, accuracy, and alignment with specific educational standards. Let's break down why this is non-negotiable.
First, consider the pilot study involving 27 students using automated essay scoring software. While the technology showed promise, it also revealed glaring gaps.
Without instructor oversight, the system struggled to account for unique linguistic expressions or cultural contexts. This isn't just a technical limitation—it's an ethical one.
If you're relying solely on automation, you risk alienating students whose writing styles or backgrounds don't fit the algorithm's narrow parameters.
Here's the reality: automated systems are trained on data, but that data isn't always representative. For example, if you're grading essays for the Malaysian University English Test (MUET), the software mightn't accurately reflect the specific standards and expectations of that exam. Human graders, on the other hand, can interpret these nuances and adjust accordingly. Without their intervention, you're leaving students at the mercy of a system that mightn't fully understand their work.
- Human graders' reliability varies, but their oversight ensures consistency and fairness.
- Automated systems lack cultural sensitivity, which can lead to biased or inaccurate evaluations.
- Specific standards like MUET require human expertise to align grading with local expectations.
Another critical issue is feedback. Automated systems can provide instant scores, but they often fall short when it comes to offering meaningful, actionable feedback—especially for second language learners.
In large classes, where instructors are already stretched thin, this becomes a major hurdle. Human intervention is essential to bridge this gap. Without it, students miss out on the detailed guidance they need to improve their writing skills.
The bottom line? Automation can streamline the grading process, but it can't replace the human touch. You need a hybrid approach—one that leverages technology for efficiency while relying on human expertise for fairness, accuracy, and meaningful feedback. Ignoring this balance isn't just a disservice to your students; it's a failure to uphold the ethical standards of education.
Ethical Guidelines
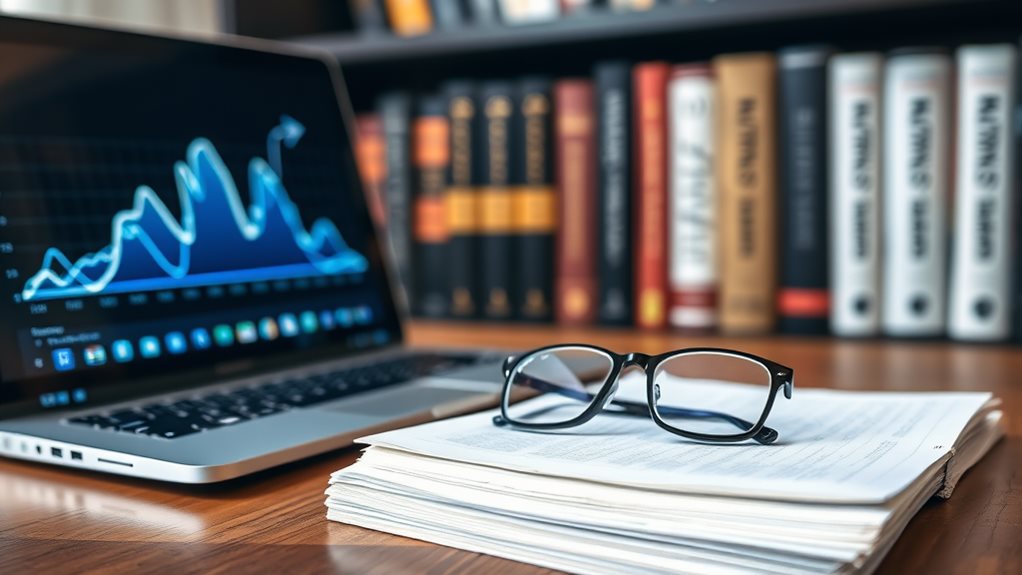
Automated essay grading (AEG) systems are transforming education, but they come with ethical considerations you can't afford to ignore. As an educator or institution, you're likely drawn to the efficiency and scalability of AEG, but you must also grapple with the ethical implications of relying on algorithms to assess student work. Let's break down the key ethical guidelines you need to consider to ensure fairness, transparency, and accountability.
1. Bias and Fairness
AEG systems are only as unbiased as the data they're trained on. If the training data reflects historical biases—such as favoring certain writing styles, dialects, or cultural references—the system may unfairly disadvantage certain groups of students. For example:
- A system trained primarily on essays from native English speakers might penalize non-native speakers for grammatical structures that are correct in their dialect.
- Essays that deviate from conventional formats or express unconventional ideas might be scored lower, stifling creativity.
To address this, you must:
- Ensure the training data is diverse and representative of all student populations.
- Regularly audit the system for bias and adjust the algorithms as needed.
- Provide human oversight to review flagged or borderline cases.
2. Transparency and Explainability
Students and educators deserve to understand how an AEG system arrives at its scores. If the grading process feels like a "black box," it can erode trust and leave students feeling powerless.
For instance:
- A student who receives a low score without clear feedback won't know how to improve.
- Educators may struggle to justify grades to parents or administrators if the system's logic isn't transparent.
To build trust, you should:
- Use systems that provide detailed, actionable feedback alongside scores.
- Ensure the algorithms are explainable, so educators can understand the reasoning behind each score.
- Offer training for educators on how to interpret and supplement AEG feedback.
3. Privacy and Data Security
AEG systems rely on vast amounts of student data, including essays, personal information, and sometimes even biometric data (e.g., keystroke patterns). This raises serious privacy concerns:
- Who owns the data—students, schools, or the AEG provider?
- How is the data stored, and who's access to it?
- Could the data be used for purposes beyond grading, such as profiling or marketing?
To protect student privacy, you must:
- Choose AEG providers with robust data security measures and clear privacy policies.
- Obtain informed consent from students and parents before using their data.
- Regularly review and update data protection protocols to comply with evolving regulations.
4. Impact on Learning and Creativity
AEG systems often prioritize quantifiable metrics like grammar, structure, and word choice. While these are important, they can inadvertently discourage creativity and critical thinking. For example:
- A student who writes a thought-provoking but unconventional essay might receive a lower score than one who follows a formulaic structure.
- Over-reliance on AEG might lead educators to focus on teaching to the algorithm rather than fostering deeper learning.
To mitigate this, you should:
- Use AEG as a supplementary tool, not a replacement for human grading.
- Encourage students to experiment with different writing styles and ideas, even if they don't align perfectly with the system's criteria.
- Regularly evaluate the impact of AEG on teaching practices and student outcomes.
5. Accountability and Oversight
When an AEG system makes a mistake—and it will—who is accountable? Is it the developer, the educator, or the institution? Without clear accountability, errors can go unchecked, harming students' academic progress.
For instance:
- A flawed algorithm might consistently misgrade essays on a specific topic, disadvantaging students who choose that subject.
- A technical glitch could result in incorrect scores being recorded, affecting students' grades and future opportunities.
To ensure accountability, you must:
- Establish clear protocols for identifying and correcting errors.
- Provide students with avenues to appeal their grades and request human review.
- Regularly evaluate the system's performance and make adjustments as needed.
Key Takeaways
- Bias and Fairness: Ensure the system is trained on diverse data and audited for bias.
- Transparency: Use systems that provide clear feedback and explainable logic.
- Privacy: Protect student data with robust security measures and informed consent.
- Learning Impact: Balance AEG with human grading to foster creativity and critical thinking.
- Accountability: Establish protocols for error correction and oversight.
Balancing Efficiency
Balancing Efficiency
When you're considering automated essay grading, efficiency is often the first thing that comes to mind. And for good reason—AI-powered systems like Automated Essay Scoring (AES) promise to save time, reduce costs, and deliver consistent results.
But here's the thing: efficiency isn't just about speed. It's about how you balance that speed with the quality of feedback and the impact on student learning.
Let's break it down. AES systems can grade essays in seconds, far outpacing human graders. For example, a pilot study with 27 students showed that AI could significantly cut grading time and costs. That's a game-changer for educators juggling large classes or tight deadlines.
But here's the catch: while AES delivers immediate scoring, the depth of feedback mightn't always match what a human grader can provide.
- Immediate Feedback: AESF (Automated Essay Scoring and Feedback) systems provide instantaneous scoring, which is ideal for students who need quick insights to improve their writing.
- Consistency: Algorithms don't get tired or biased, ensuring 100% reliability in grading.
- Scalability: AES can handle large-scale assessments effortlessly, making it a practical solution for institutions with limited resources.
However, efficiency gains aren't automatic. For second language learners or students in large classes, the feedback might still feel impersonal or insufficient.
That's where you, as an educator, come in. You need to evaluate whether the speed of AES aligns with your students' needs. Are they getting the kind of feedback that helps them grow? Or are they just getting a score?
The key is to use AES as a tool, not a replacement. Pair it with targeted human feedback to address nuanced issues like argument structure or cultural context. This hybrid approach ensures you're not sacrificing quality for speed.
Future of AI Grading
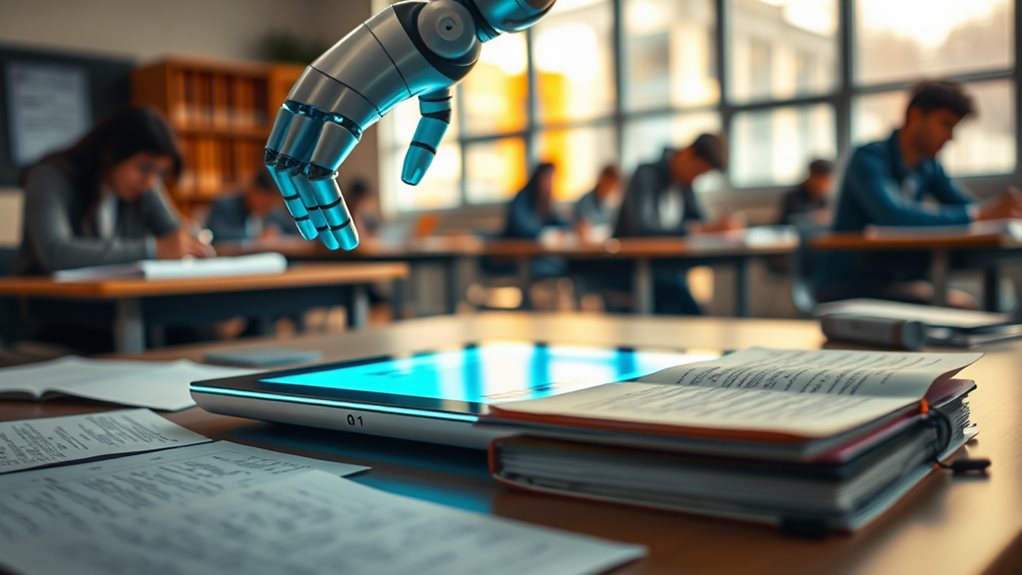
The future of AI grading isn't just about efficiency—it's about redefining how you approach education while addressing critical ethical challenges.
As AI systems evolve, you'll see them become more sophisticated in handling nuanced language and context, but human oversight will remain indispensable.
Why? Because AI, no matter how advanced, still struggles with understanding cultural subtleties, emotional tone, and the creativity that often defines exceptional student work.
Here's what you need to prepare for:
- Increased reliance on hybrid models: AI will handle the bulk of grading, but educators will step in to review edge cases, ensuring fairness and accuracy.
- Real-time feedback loops: Students will receive immediate, actionable feedback, allowing them to iterate and improve their work faster than ever before.
- Personalized learning paths: AI will identify patterns in student performance, enabling you to tailor instruction to individual needs.
But with these advancements come challenges.
Algorithmic bias is a persistent concern, especially in diverse educational settings.
For example, in the Malaysian MUET context, AI systems must be trained to align with specific linguistic and cultural nuances to avoid disadvantaging certain groups.
This isn't just a technical issue—it's an ethical imperative.
You'll also need to navigate the balance between efficiency and human connection.
While AI can grade essays in seconds, it can't replicate the mentorship and encouragement that come from a teacher's feedback.
The key is to use AI as a tool to free up your time, so you can focus on building meaningful relationships with your students.
The pilot study involving 27 students using AI for business law essay grading highlights the potential of this technology.
Students wrote more essays and received immediate feedback, which accelerated their learning.
But the study also underscores the importance of continuous educator training.
As AI evolves, so must your skills in leveraging it effectively.
The future of AI grading is bright, but it's not without its pitfalls.
Your role as an educator will shift from being a grader to being a guide, ensuring that AI enhances—not replaces—the human elements of teaching.
Stay informed, stay adaptable, and you'll be ready to lead in this new era of education.
Questions and Answers
What Are the Ethical Considerations in an Essay?
You must consider plagiarism detection to ensure originality, address bias mitigation to promote fairness concerns, and maintain human oversight to uphold ethical standards, balancing technology with principles of equity and integrity in essay evaluation.
What Is the Automated Essay Scoring Model?
You'll find automated essay scoring models use algorithms to assess essays, prioritizing accuracy and reliability. However, you must consider potential bias and fairness issues, as these systems may favor certain writing styles or penalize creative expression.