Automated essay scoring (AES) enhances formative assessment by providing immediate, objective feedback to students, enabling quicker revisions and improved engagement. It reduces grading time for educators, allowing them to focus on personalized instruction. AES systems predict standardized test performance effectively and scale across diverse class sizes, making them cost-effective and accessible. However, challenges like cultural biases and implementation barriers must be addressed to ensure fairness and equity. By integrating AES with adaptive learning tools and refining algorithms, you can maximize its potential for formative assessment. Exploring further reveals strategies to optimize its use and address emerging challenges in education.
Benefits of Automated Essay Scoring in Education
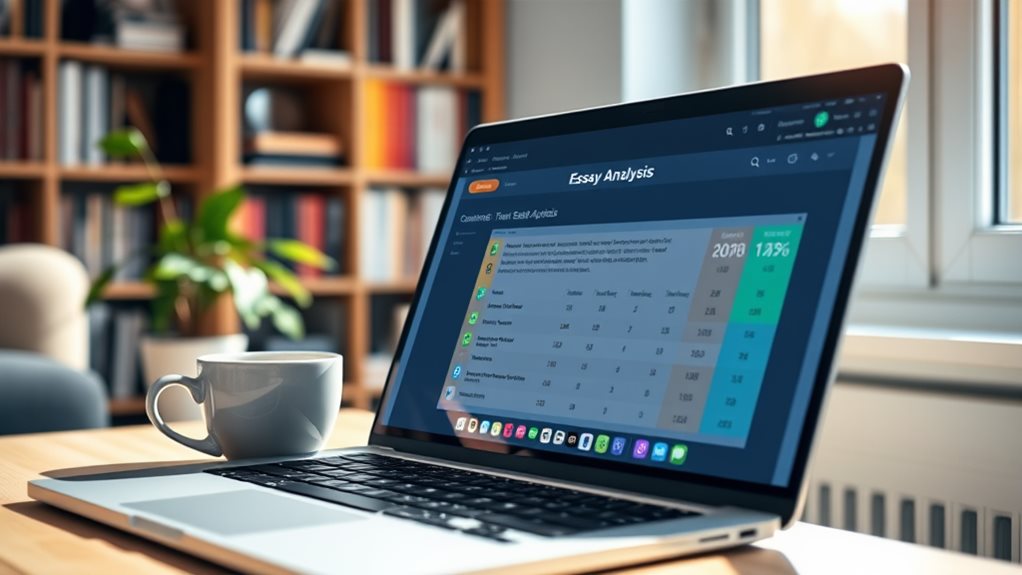
Automated essay scoring (AES) is revolutionizing how educators approach formative assessment, and the benefits are too significant to ignore. If you're looking to streamline your grading process while enhancing student outcomes, AES is a game-changer. Let's break down why this technology is becoming indispensable in modern education.
First, AES provides immediate feedback to students, which is critical for accelerating the learning process. Imagine a student submitting an essay and receiving detailed insights within minutes—not days or weeks. This immediacy allows them to identify weaknesses and adjust their writing strategies in real time. For you, this means students are better prepared for future assignments and standardized tests, reducing the need for last-minute interventions.
- Faster feedback loops: Students can revise and improve their work before bad habits solidify.
- Increased engagement: Immediate results keep students motivated and invested in their progress.
- Data-driven insights: AES highlights patterns in student performance, helping you tailor instruction more effectively.
Second, AES drastically reduces grading time for educators. If you've ever spent hours poring over stacks of essays, you know how draining it can be. With AES, that time is freed up, allowing you to focus on what truly matters—providing individualized instruction and fostering deeper learning. This isn't just about convenience; it's about maximizing your impact as an educator.
Third, studies consistently show that AES is highly effective in predicting student performance on standardized writing tests. This isn't just a theoretical benefit—it's a practical tool for identifying student strengths and weaknesses early on. For example, if AES flags recurring issues with sentence structure or argument development, you can address these areas before they become entrenched. This predictive capability gives you a clear roadmap for intervention, ensuring no student falls through the cracks.
- Consistent scoring criteria: AES applies the same standards to every essay, eliminating the variability that often comes with human grading.
- Fairness and equity: Students are evaluated objectively, reducing potential biases.
- Scalability: Whether you're teaching 20 students or 200, AES ensures every essay is assessed with the same rigor.
Finally, the cost-effectiveness of AES makes it accessible for schools and institutions of all sizes. Traditional grading methods often require significant resources, especially for large classes. AES, on the other hand, offers a scalable solution that doesn't compromise on quality. This affordability means you can implement frequent formative assessments without breaking the budget, giving students more opportunities to practice and improve.
- Frequent assessments: Students can write and revise more often, building skills incrementally.
- Resource allocation: Savings on grading can be redirected to other critical areas, like professional development or classroom resources.
- Accessibility: Even underfunded schools can leverage AES to provide high-quality feedback to students.
In short, AES isn't just a tool—it's a strategic advantage for educators and students alike. By integrating this technology into your formative assessment practices, you're not only saving time but also empowering students to reach their full potential. The question isn't whether you can afford to use AES—it's whether you can afford not to.
Case Studies: AES Implementation in China and the U.S
Let's dive into the fascinating case studies of AES implementation in China and the U.S. These real-world examples reveal how cultural and systemic differences shape the adoption and impact of automated essay scoring in education.
AES in China: A Deep Dive
In China, AES was implemented within a highly structured educational system, where standardized testing and academic performance are paramount. The case study highlighted several key findings:
- Impact on Learning Outcomes: AES significantly improved student writing skills by providing immediate, consistent feedback. Students were able to identify and correct errors faster, leading to measurable gains in essay quality over time.
- Technology Acceptance: Teachers initially hesitated, fearing AES might replace their roles. However, they soon embraced it as a tool to reduce grading workload and focus on personalized instruction. Students, accustomed to technology-driven learning, adapted quickly and appreciated the instant feedback.
- Cultural Influence: The collectivist culture in China played a role in the smooth adoption of AES. Students were motivated to improve collectively, and teachers viewed AES as a way to enhance the overall quality of education.
AES in the U.S.: A Contrasting Perspective
In the U.S., AES was implemented in a more decentralized educational system, with a focus on individualized learning and creativity. The results were strikingly different:
- Impact on Learning Outcomes: While AES improved efficiency in grading, some educators raised concerns about its ability to assess creativity and critical thinking. Students benefited from faster feedback but occasionally felt their unique writing styles were undervalued.
- Technology Acceptance: Teachers in the U.S. were more skeptical about AES, questioning its fairness and accuracy. Students, on the other hand, appreciated the reduced grading time but expressed mixed feelings about the impersonal nature of automated feedback.
- Cultural Influence: The individualistic culture in the U.S. influenced the perception of AES. Students valued personalized feedback from teachers, and educators emphasized the importance of human judgment in assessing nuanced aspects of writing.
Cross-Cultural Insights
The comparison between China and the U.S. underscores how cultural and systemic factors shape the adoption and effectiveness of AES:
- Educational Systems: China's centralized system facilitated uniform implementation, while the U.S.'s decentralized approach led to varied outcomes.
- Student Populations: Chinese students, accustomed to rigorous testing, adapted seamlessly to AES. U.S. students, with a focus on creativity, required more nuanced integration.
- Challenges and Benefits: Both countries faced challenges, such as teacher skepticism and concerns about fairness. However, the benefits—improved efficiency, faster feedback, and enhanced learning outcomes—were universally recognized.
Key Takeaways
- Cultural Context Matters: AES implementation must align with the cultural and educational values of the student population.
- Teacher Involvement is Crucial: Addressing teacher concerns and involving them in the process ensures smoother adoption.
- Balancing Efficiency and Personalization: While AES excels in efficiency, integrating it with human feedback preserves the personal touch students value.
Cross-Cultural Insights on AES Effectiveness
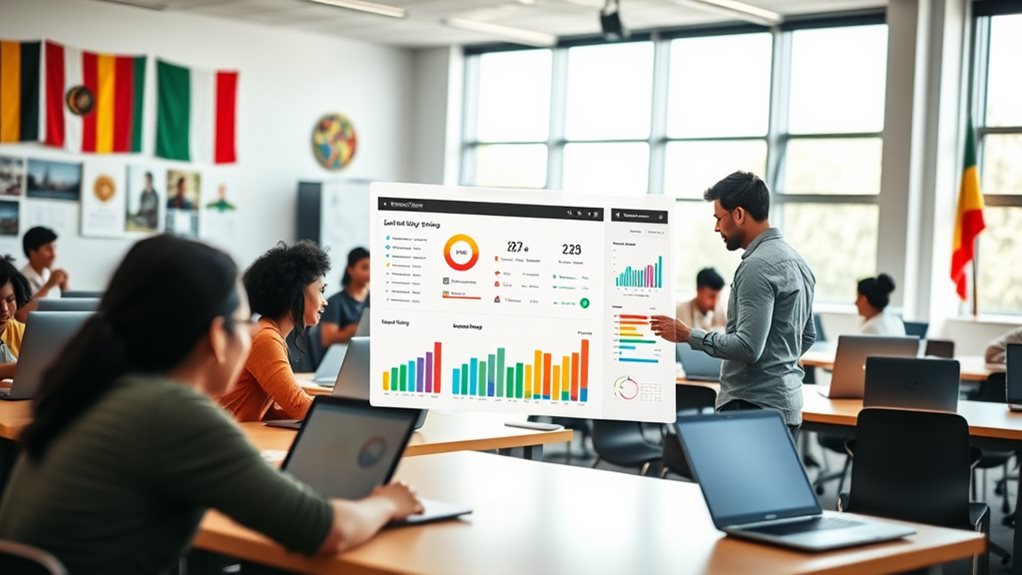
Automated Essay Scoring (AES) systems have revolutionized formative assessment, but their effectiveness isn't universal—it varies significantly across cultures. If you're implementing AES in diverse educational settings, understanding these cross-cultural nuances is critical. Let's dive into what makes AES work—or falter—in different cultural contexts.
Why Culture Matters in AES Effectiveness
AES systems are often trained on datasets from specific regions, primarily English-speaking countries. This means they're optimized for certain linguistic structures, writing styles, and cultural references. When deployed in non-Western or multilingual environments, these systems can struggle to accurately assess essays.
For example:
- Language Nuances: Idioms, metaphors, and culturally specific expressions may be misinterpreted by AES algorithms. A phrase that's common in Indian English might be flagged as unclear or incorrect in a system trained on American English.
- Writing Conventions: In some cultures, indirect communication is valued, while others prioritize directness. AES systems calibrated for direct writing styles might penalize essays that use subtlety or storytelling, even if they're culturally appropriate.
- Cultural References: Essays that draw on local history, literature, or traditions may not align with the system's training data, leading to lower scores despite their quality.
Real-World Examples of Cross-Cultural Challenges
Consider these scenarios:
- In Japan, students often use a narrative style that builds gradually toward a conclusion. AES systems trained on Western essays, which typically emphasize a clear thesis upfront, might score these essays lower for structure.
- In Nigeria, where English is heavily influenced by local languages, students might use unique grammatical constructions. An AES system might flag these as errors, even though they're valid in Nigerian English.
- In the Middle East, essays often include religious or cultural references that aren't part of the system's training data. This can lead to misinterpretation or undervaluation of the content.
How to Adapt AES for Cross-Cultural Use
If you're working with AES in a multicultural context, here's how you can enhance its effectiveness:
- Localize Training Data: Incorporate essays from the target culture into the system's training dataset. This helps the algorithm recognize and value culturally specific writing styles and references.
- Customize Rubrics: Adjust scoring criteria to align with local educational standards and cultural expectations. For example, if storytelling is a valued skill in a particular culture, ensure the rubric reflects that.
- Provide Contextual Feedback: Use AES as a tool to highlight areas for improvement, but pair it with human feedback to address cultural nuances that the system might miss.
- Test and Iterate: Continuously evaluate the system's performance in the target culture. Gather feedback from educators and students to identify and address biases or gaps.
The Bigger Picture: AES as a Tool, Not a Replacement
While AES can streamline formative assessment, it's not a one-size-fits-all solution. Its effectiveness hinges on how well it's adapted to the cultural context. By acknowledging and addressing these cross-cultural challenges, you can harness the power of AES to support diverse learners—without compromising fairness or accuracy.
Addressing Bias in Automated Writing Evaluation
When you're implementing automated essay scoring (AES) for formative assessment, addressing bias is non-negotiable. Bias in AES systems isn't just a theoretical concern—it's a real-world issue that can disproportionately impact English Language Learners (ELLs) and other marginalized student groups. Let's break down what you need to know to ensure your system is as fair and effective as possible.
The Reality of Bias in AES
Studies have shown that AES systems can inadvertently replicate the same biases present in human scoring. For example, ELLs often underperform compared to their non-ELL peers on essays and state writing tests, and AES systems can mirror these disparities. This isn't just a technical glitch—it's a systemic issue tied to how these systems are trained and validated.
- Training Data Matters: A lack of representation of ELLs in the training data used to develop AES systems is a major contributor to bias. If the system isn't exposed to enough examples of ELL writing, it may struggle to accurately assess their work.
- Replicating Human Bias: Research on MI Write, an AES system, found that while it didn't introduce unique bias relative to human scores, it did replicate existing biases in predicting state test performance for ELLs.
Why This Matters for Formative Assessment
Formative assessment is all about providing actionable feedback to help students improve. If your AES system is biased, it could:
- Mislead ELLs about their writing strengths and weaknesses.
- Reinforce systemic inequities by undervaluing their work.
- Erode trust in the system among educators and students alike.
How to Mitigate Bias in Your AES System
- Diversify Training Data: Ensure your system is trained on a robust dataset that includes a wide range of ELL writing samples. This helps the system learn to recognize and fairly evaluate diverse linguistic patterns.
- Conduct Bias Audits: Regularly test your system for bias by comparing its scores with human scores across different student groups. Look for discrepancies that could indicate systemic issues.
- Use Multiple Metrics: Don't rely solely on automated scores. Combine AES with human evaluation or other formative assessment tools to provide a more balanced perspective.
- Monitor Long-Term Outcomes: Research has shown that bias in AES systems can have long-term consequences, such as inaccurate predictions of future test performance. Track how your system's scores correlate with student outcomes over time.
The Bigger Picture
Bias in AES isn't just a technical problem—it's an equity issue. When you address bias, you're not just improving your system; you're creating a fairer learning environment for all students. And in today's educational landscape, that's not just a nice-to-have—it's a must.
Future Applications and Challenges of AES Technology
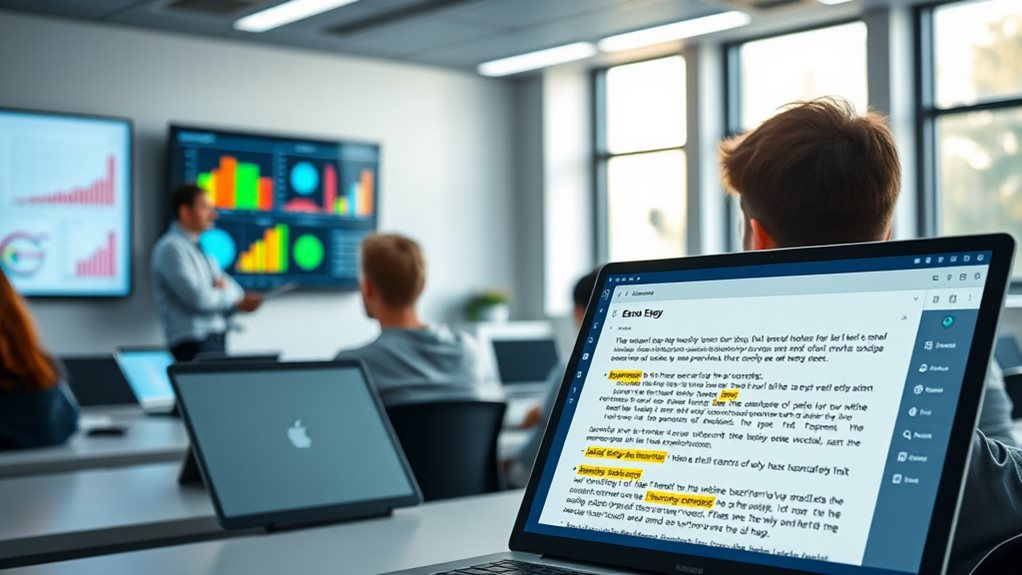
The future of Automated Essay Scoring (AES) is brimming with potential, but it's not without its hurdles. As you look ahead, you'll see how this technology is poised to revolutionize formative assessment—if we can navigate the challenges effectively. Let's break it down.
Future Applications: Personalized Learning at Scale
Imagine AES systems that don't just score essays but actively guide students toward improvement. By integrating with adaptive learning platforms, AES can provide personalized feedback tailored to each student's unique needs.
For example:
- A student struggling with thesis statements could receive targeted exercises and examples to strengthen that skill.
- Another excelling in argumentation but weak in grammar might get prompts to refine sentence structure.
This level of personalization isn't just a pipe dream—it's within reach, thanks to advancements in AI and natural language processing (NLP). Large language models (LLMs) are already showing promise in analyzing nuanced aspects of writing, such as creativity and complex argumentation, which traditional AES systems often miss.
Challenges: Bias and Equity
But here's the catch: AES systems are only as fair as the data they're trained on. Studies have shown that these systems can replicate biases present in human scoring, disadvantaging students from diverse linguistic or cultural backgrounds.
For instance:
- Essays written in non-standard dialects might be penalized unfairly.
- Cultural references unfamiliar to the algorithm could lead to lower scores.
To ensure equitable assessment, developers must prioritize bias mitigation strategies, such as diversifying training datasets and implementing fairness checks. This isn't just a technical challenge—it's an ethical imperative.
Expanding Access: Overcoming Barriers
Another critical challenge is accessibility. While AES has the potential to democratize feedback, its implementation is often hindered by cost, infrastructure limitations, and varying levels of digital literacy.
Consider this:
- Schools in underfunded districts may lack the resources to adopt AES technology.
- Teachers and students unfamiliar with digital tools might struggle to integrate AES into their workflows.
To bridge this gap, stakeholders must invest in affordable solutions, provide robust training, and ensure that AES tools are user-friendly. Only then can we achieve equitable access across all educational settings.
The Road Ahead: Research and Impact
Finally, we need more research to fully understand the long-term impact of AES on student learning outcomes. Questions remain:
- How does AES influence student motivation and engagement?
- What role do teachers play in a classroom where AES provides instant feedback?
These aren't just academic questions—they're practical concerns that will shape the future of education. By addressing them, we can ensure that AES enhances, rather than replaces, the human element of teaching.
The future of AES is bright, but it's up to us to navigate the challenges and unlock its full potential. The time to act is now—because the students of tomorrow deserve nothing less.
Questions and Answers
What Are the Advantages of Automated Essay Scoring?
You'll gain time savings and cost efficiency with automated essay scoring. It ensures objective grading, immediate feedback, and consistent scoring. It's ideal for large scale assessment, offering unbiased evaluation, scalability potential, robust data analysis, and enhanced student engagement.
Can an Essay Be a Formative Assessment?
An essay can be a formative assessment if it provides actionable insights through essay feedback, encourages student reflection, and focuses on skill development. It helps address learning goals, reduces teacher workload, and improves writing through an iterative, process-focused approach.
What Is the AES Scoring System?
The AES scoring system uses NLP and ML to grade essays. You'll find it evaluates grammar, organization, and style, but concerns like AES reliability, bias, and fairness persist. Its development focuses on improving feedback and addressing ethical limitations.
What Is the Automated Essay Scoring Model?
You'll find automated essay scoring models use feature engineering, model types like regression or neural networks, and grading rubrics. They rely on data needs, accuracy rates, and human feedback but face bias concerns, ethical issues, and system limitations.